What if I told you that 70% of today’s jobs won’t exist in 15 years? Not because of economic collapse, but because AI and robotics will simply do them better, faster, cheaper, and safer than humans ever could. The signs are already here – from self-checkout lanes to AI writing assistants to automated factories.
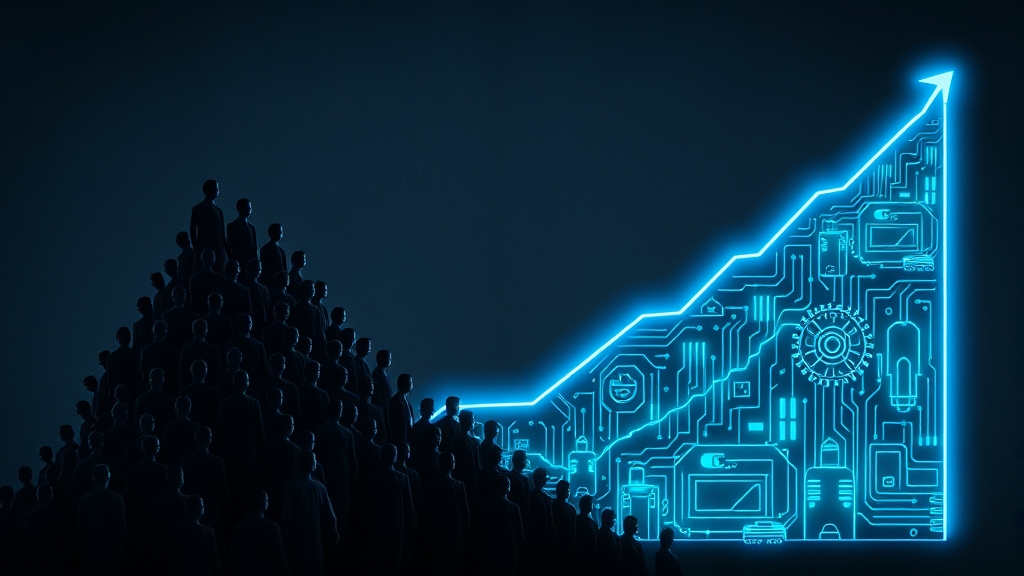
This technological revolution threatens both physical and knowledge work simultaneously. Like a tidal wave reshaping the shoreline, automation is fundamentally changing our economic landscape. As we approach post-labor economics, we must ask: what happens when human labor no longer anchors our economy? The answer may transform society more profoundly than anything since the Industrial Revolution.
The Four Horsemen of Job Extinction
When automation threatens jobs, it’s never just about cost-cutting. Four specific criteria determine whether a machine will replace human workers in any industry. I call these the Four Horsemen of Job Extinction: better, faster, cheaper, and safer. When technology satisfies all four criteria simultaneously, it creates an economic inevitability that businesses simply cannot ignore.
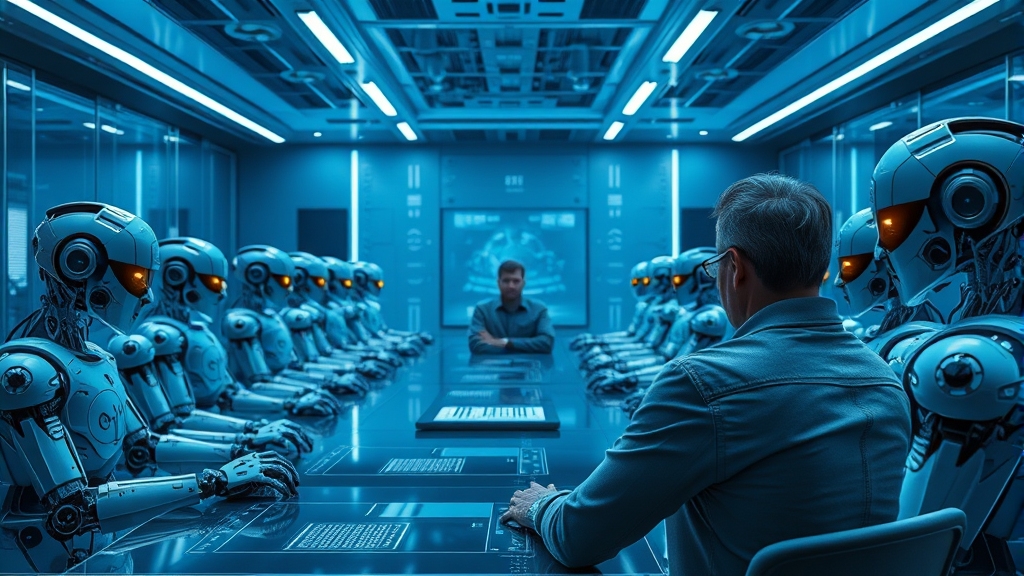
Let’s examine what makes these criteria so powerful. The economic formula is straightforward: when machines consistently outperform humans across all four dimensions, keeping human workers becomes economically irrational. This pattern has repeated throughout technological history, from steam shovels replacing manual diggers to AI now replacing knowledge workers.
The first criterion—”better”—means machines consistently produce higher quality outputs than humans. In coding, advanced AI models now outperform human coders in creating efficient, error-free code. Picture a software development team working for days on a complex algorithm, making revisions after finding bugs during testing, while an AI system delivers a flawless solution in minutes. These AI systems already operate above the 90th percentile of human performance—imagine a room with 100 programmers where the AI writes better code than 90 of them. In manufacturing, robots create products with precision humans simply cannot match. Even in creative fields, AI now generates designs, music, and written content that rivals human-created work in blind tests.
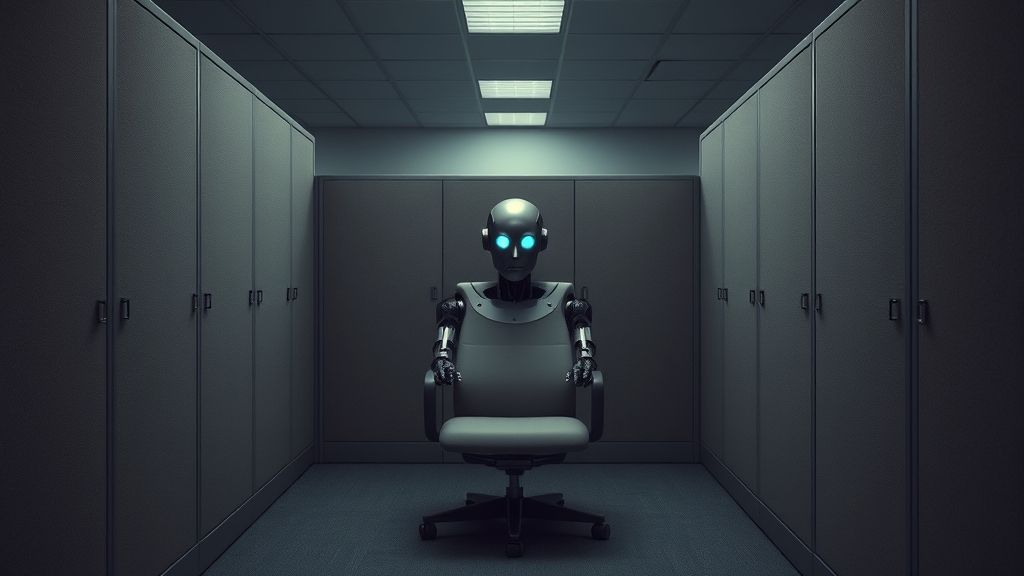
The speed advantage machines have over humans—our second criterion—creates an insurmountable competitive gap in the marketplace. This speed differential transforms entire workflows and business models. Visualize a financial analyst spending weeks analyzing market trends, compiling reports, and making projections, while an AI system processes millions of transactions, identifies patterns, and delivers actionable insights before the analyst even finishes gathering data. In warehouses, a single automated system moves inventory and processes orders at speeds requiring dozens of human workers to match. When businesses can complete tasks in seconds rather than hours, the economic pressure to adopt automation becomes overwhelming.
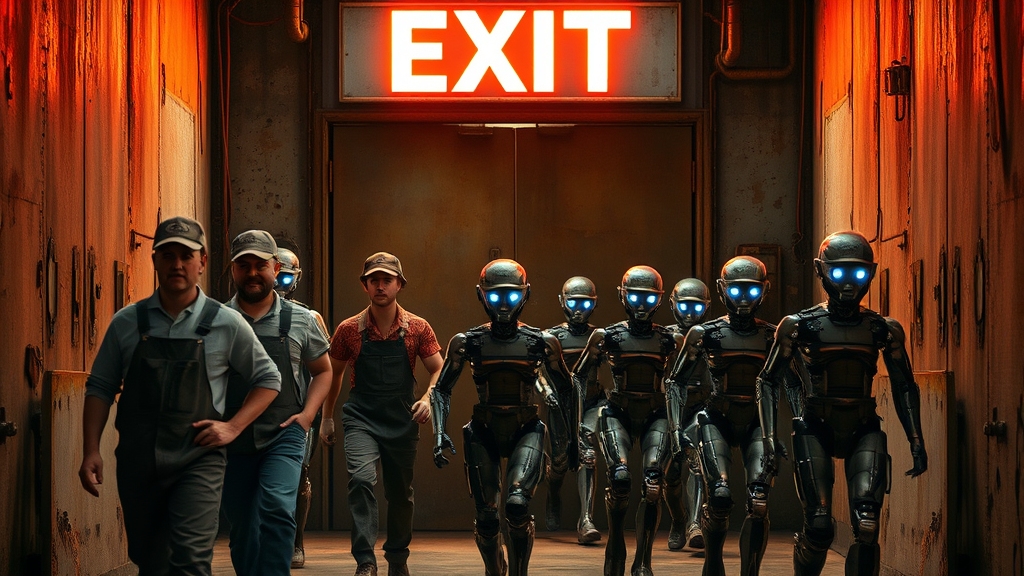
Beyond speed, the “cheaper” criterion extends far beyond hourly wages to the total cost of employment. When comparing these costs to automation, the difference becomes stark. A factory robot operates 24/7 with minimal downtime—no health insurance, retirement benefits, or paid vacation required. It doesn’t need management oversight or HR support. It doesn’t make costly mistakes resulting in wasted materials or product recalls. Over a 5-10 year period, the total cost comparison between automation and human labor creates economic pressure that businesses cannot ignore.
Safety advantages—our fourth criterion—directly translate to reduced insurance costs, fewer workplace incidents, and lower legal liabilities. Self-driving vehicles demonstrate fewer fatal accidents per mile than human drivers. Robots handle dangerous materials without exposure risk. AI systems make consistent decisions without the bias, fatigue, or emotional variables affecting human judgment. For businesses facing increasing regulatory requirements, automation provides a reliable way to enforce safety and compliance standards.
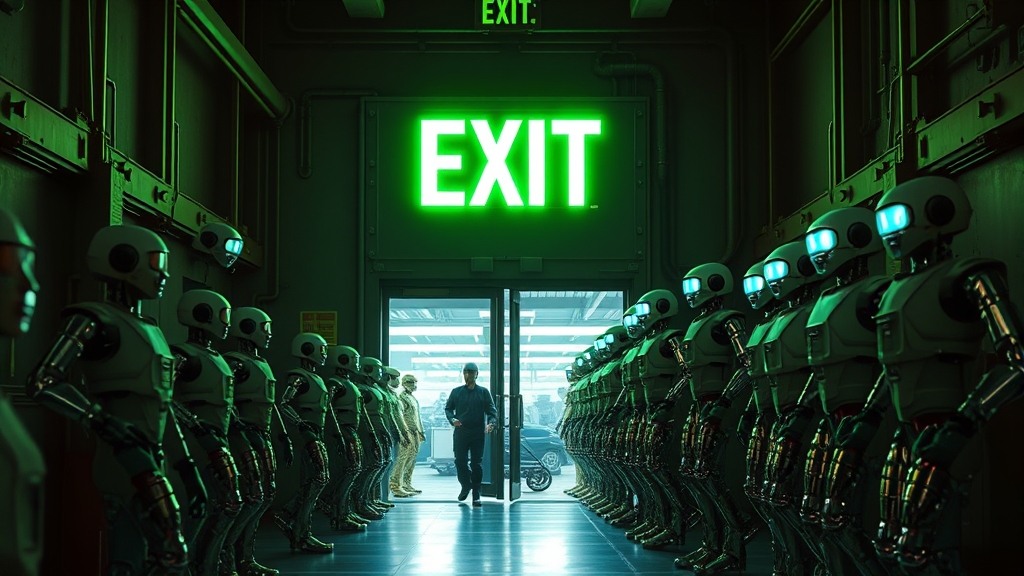
What’s particularly concerning is how these criteria now apply across increasingly diverse job categories. Initially, automation primarily affected repetitive manufacturing tasks. Now, these four criteria are being satisfied in professional fields once thought immune: legal research, medical diagnosis, financial analysis, and creative work. When machines perform better, faster, cheaper, and safer, market forces push businesses toward automation regardless of the industry.
The economic formula works like a tipping point. Imagine running a business where competitors implement automation that produces better results, finishes projects in half the time, costs 70% less, and eliminates workplace accidents. How long could you continue employing humans for the same tasks and remain competitive? This creates a situation where individual business decisions, each rational on their own, collectively transform entire industries.
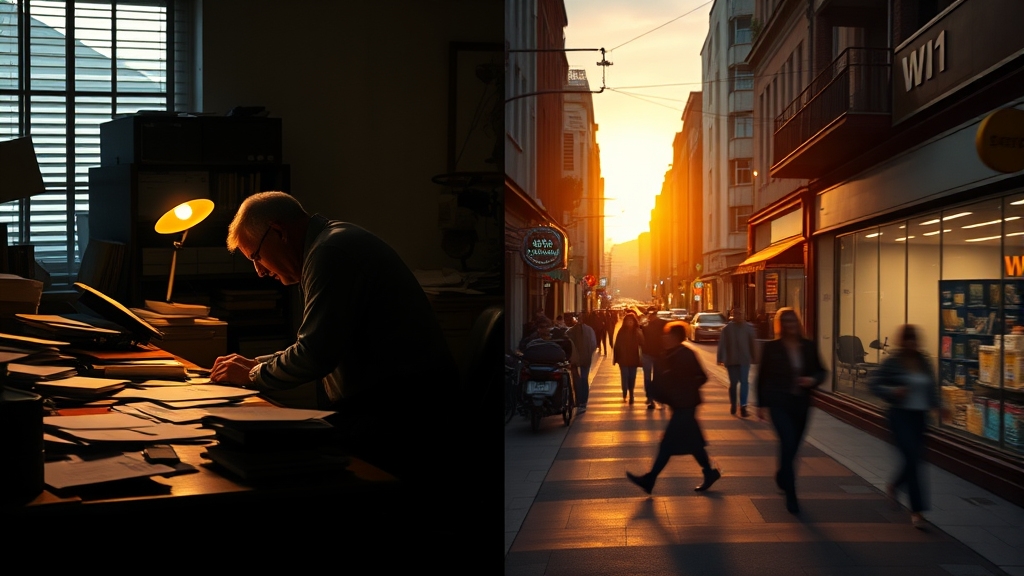
What makes the current wave of automation different from previous technological shifts is the breadth of capabilities being developed simultaneously. Artificial intelligence combined with advanced robotics targets both physical and mental labor, developing capabilities that exceed human performance across the entire spectrum of work. Unlike the steam engine, which replaced muscle power but created demand for cognitive abilities, today’s systems outperform humans in both domains.
For workers, this presents an uncomfortable reality. Skills that took years to develop can become economically obsolete because machines now deliver those same skills more effectively. This is happening in fields as diverse as radiological analysis, legal document review, customer service, and creative work.
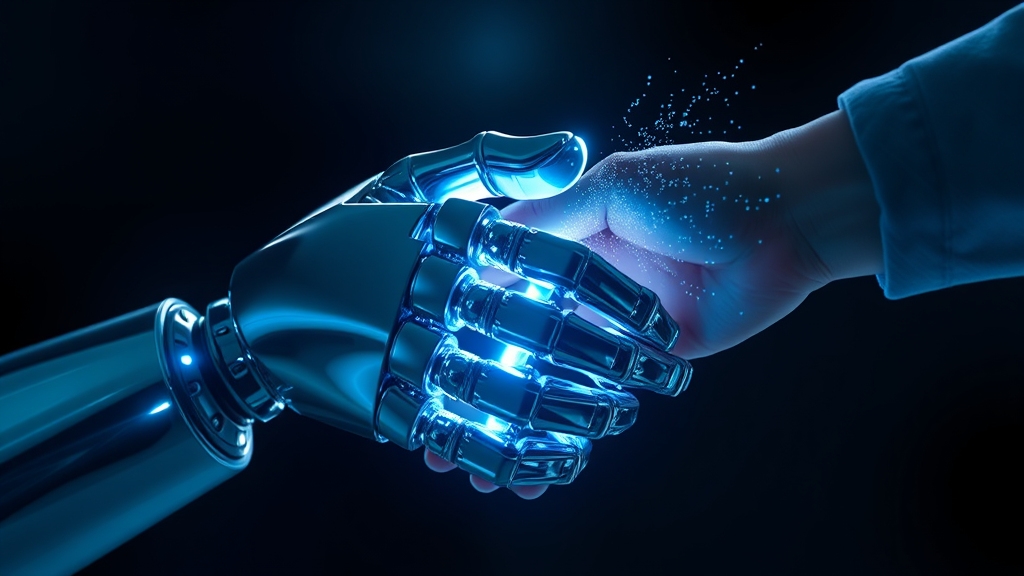
The four criteria create a clear framework for predicting which jobs will be automated next. Any occupation where machines approach human performance while offering speed, cost, and safety advantages is endangered. The timeline may vary, but the economic outcome becomes essentially inevitable once all four criteria are satisfied.
This leads us to ask: which human capabilities are machines already surpassing, and which ones will be next? Understanding the progression of machine capabilities across different domains of human skill helps us see where this automation wave is heading. Machines are conquering human skill domains one by one, following a clear pattern that reveals what’s coming.
The Human Skills Countdown
We humans have always taken pride in certain abilities we thought would forever remain uniquely ours. Our strength to build civilizations, our dexterity to create art, our intellect to solve complex problems, and our emotional connections that bind communities together. These four domains—strength, dexterity, cognition, and empathy—represent the core of human productive capability throughout history. But a sobering reality is emerging: machines are conquering these domains one by one, and they’re doing it faster than most experts anticipated.
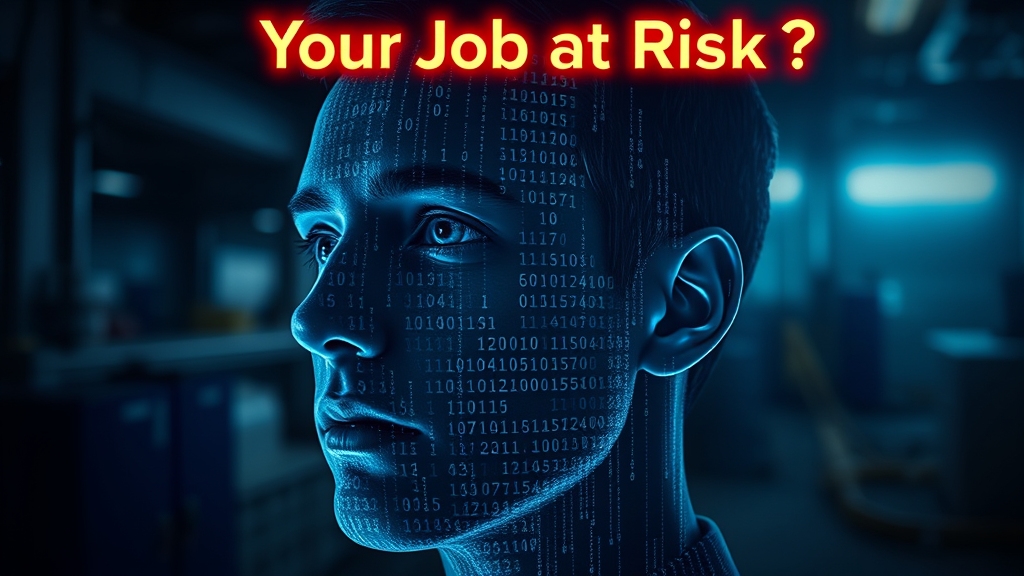
These four categories create a useful framework for understanding the progression of automation—a countdown of human comparative advantage, with machines claiming each territory in sequence. Understanding this progression helps us see where we stand now and what’s coming next in the transformation of work.
Strength was the first domain where machines completely eclipsed human capability. This transition began centuries ago but accelerated dramatically during the Industrial Revolution. Today, human strength is largely obsolete in economic terms. At the River Rouge Complex in Michigan, Ford’s manufacturing evolution tells this story perfectly. Where once hundreds of workers manually lifted and positioned heavy components, now a single hydraulic system exerts forces equivalent to those hundreds of workers. Industrial robots routinely lift and move objects weighing thousands of pounds with precision impossible for humans. In manufacturing, construction, and logistics, relying primarily on human muscle power seems almost primitive.
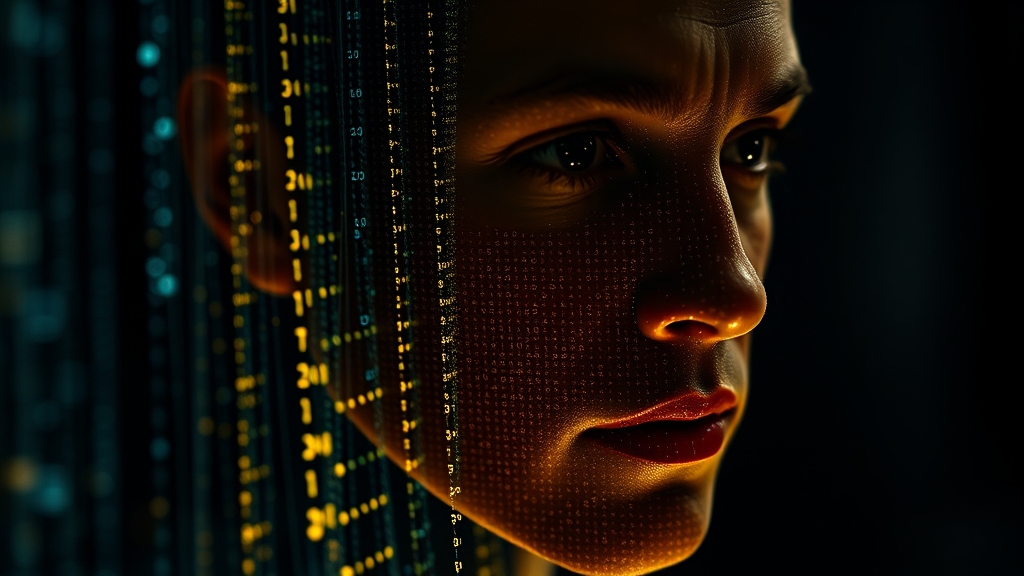
Modern automotive manufacturing demonstrates this reality. Robots lift and position entire car frames weighing over a ton, holding them with perfect stability while performing welding operations. The physical strength of these machines exceeds human capability by orders of magnitude. One industrial robot can apply consistent force equivalent to dozens of workers without fatigue or variation in performance. This strength advantage creates productivity gains that make human manual labor economically unsustainable in many contexts.
We rarely think about this first transition anymore. The replacement of human strength with machine power has become so normalized that we don’t consider it automation—it’s simply how things are done. This pattern of normalization follows each wave: what initially appears revolutionary eventually becomes unremarkable.
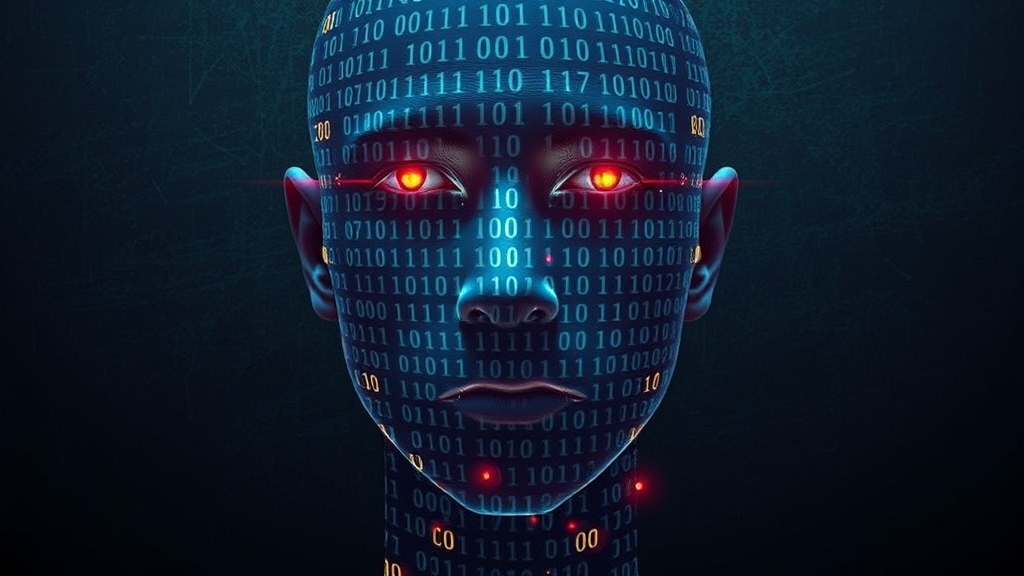
Dexterity represents the frontier that robotics has been conquering over the past decade. Historically, machines could outmuscle humans but couldn’t match our fine motor control. Tasks requiring precision manipulation—like threading a needle or handling delicate objects—remained firmly in human hands. This created a dividing line: machines handled heavy lifting while humans performed precise manipulation.
That line is now blurring. Recent breakthroughs have produced machines with dexterity approaching or exceeding human capability. Surgical robots perform procedures requiring micrometer precision that human surgeons struggle to match consistently. Assembly robots build electronic devices, handling components barely visible to the human eye. Agricultural robots pick delicate fruits without bruising them, a task once considered uniquely human.
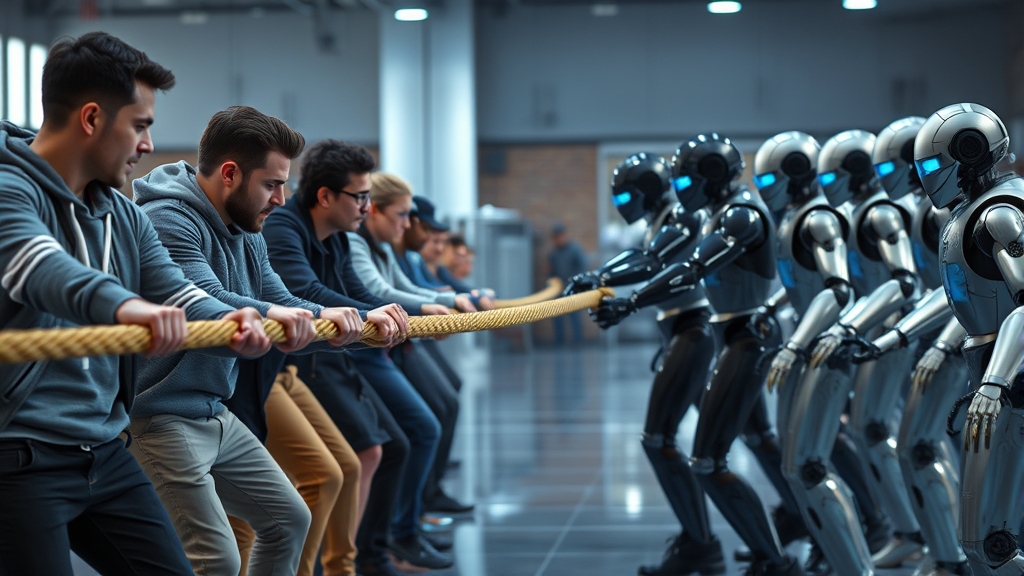
The latest robotic hands combine sophisticated sensors with advanced materials mimicking human skin’s flexibility and sensitivity. These systems identify objects by touch alone, apply precisely calibrated pressure, and perform complex manipulations previously requiring human hands. As this technology improves, the dexterity advantage that kept many manual trades safe from automation is disappearing.
This brings us to the current frontier: cognition. AI is making dramatic advances, threatening roles requiring thinking, judgment, and creative problem-solving. This phase targets the knowledge work that previous waves of automation created. When physical labor was automated, workers shifted to cognitive tasks. Now those cognitive tasks themselves are being automated.
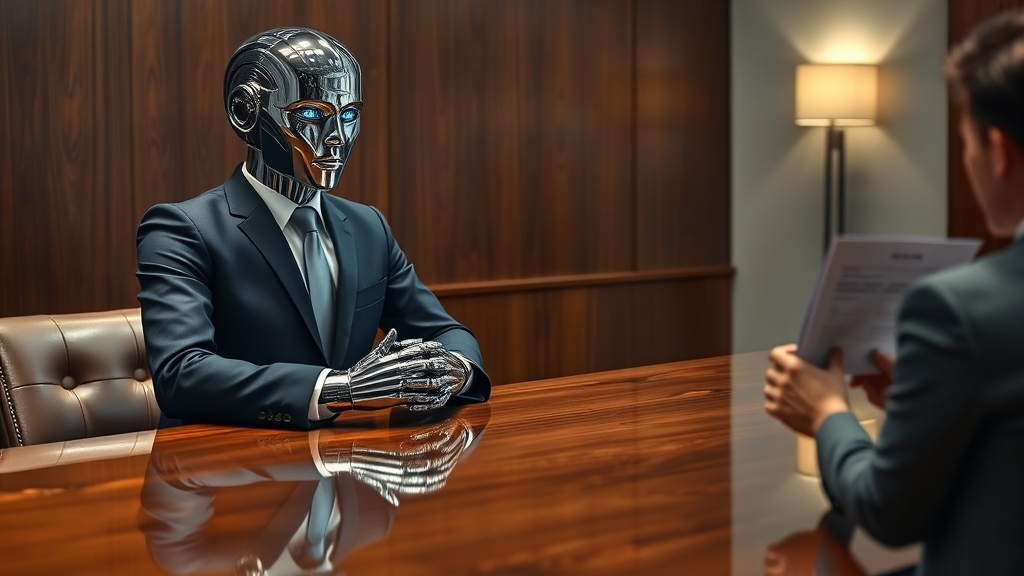
AI systems already outperform humans in specific cognitive domains. Chess and Go were early examples, but now the list includes medical diagnosis, legal document analysis, financial forecasting, and creative work. AI examining X-rays can detect early-stage lung cancer more accurately than experienced radiologists. Legal AI reviews thousands of contracts faster than human lawyers. Writing algorithms generate content that readers often cannot distinguish from human-written text.
This cognitive automation wave shows exponential progression. Early AI systems were brittle and limited. Today’s models demonstrate flexibility, learning capability, and generalization approaching human-like adaptability. Each generation shows dramatic improvements, with capabilities that would have seemed like science fiction five years earlier.
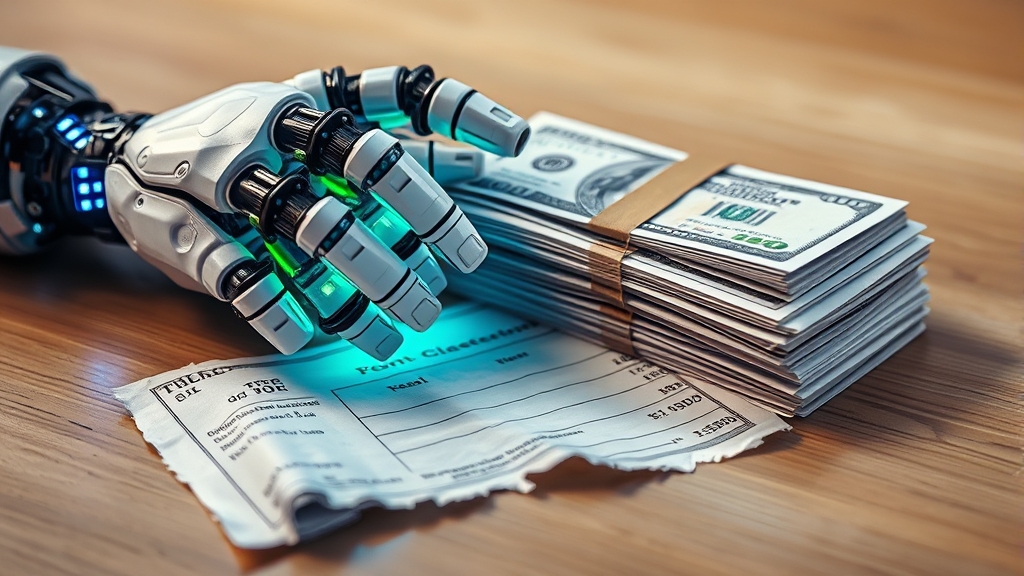
The final domain, representing humanity’s last stand against automation, is empathy and charisma—our ability to connect emotionally, build authentic relationships, and influence others through understanding their feelings. Even here, the territory faces challenges. Customer service AI recognizes emotional cues, adjusting responses to match customers’ emotional states. Digital avatars with realistic expressions mimic human emotional responses convincingly. AI companions for eldercare form relationships with users who sometimes prefer their consistency to human caregivers.
These systems don’t actually “feel” emotions. But economically, that distinction may not matter if they simulate empathetic responses convincingly enough. When an AI detects frustration, acknowledges it appropriately, and adjusts its approach, the functional difference between simulated and genuine empathy blurs.
What makes this progression troubling is its acceleration. The strength transition played out over centuries. The dexterity transition occurred over decades. The cognitive transition is happening in years. Each domain falls to automation faster than the last as technology builds upon itself.
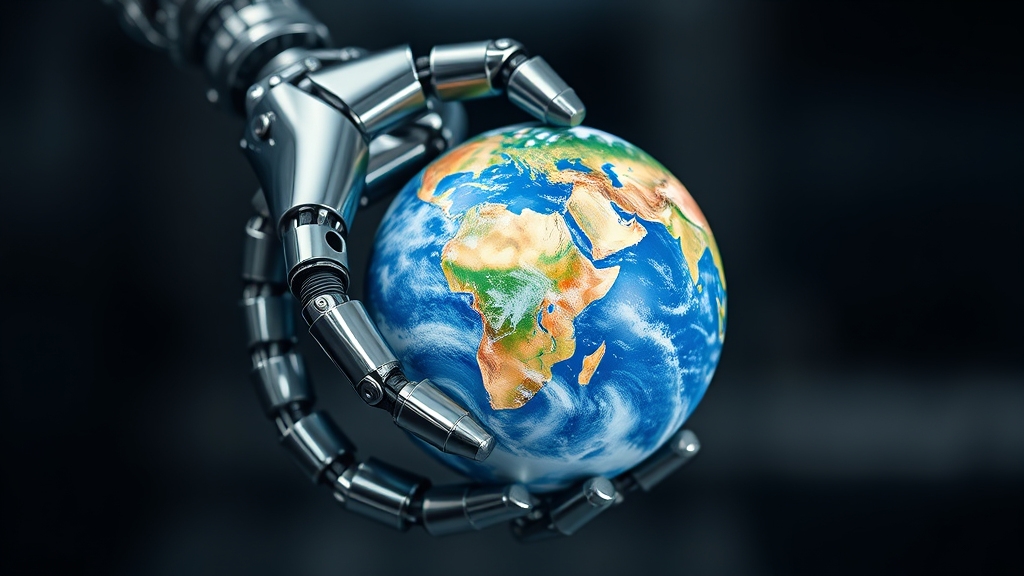
As machines match or exceed human capabilities, our understanding of work fundamentally changes. The historical pattern of technological disruption creating new jobs may not hold when machines can potentially outperform humans across all domains of productive capability. We’re witnessing the potential obsolescence of human labor as an economic input.
This creates profound implications for the social contract defining modern economies. Our economic system presumes that people exchange labor for wages to participate as consumers. When machines perform that labor more effectively across all domains, the fundamental relationship between work, income, and consumption breaks down.
The Broken Social Contract
Modern society rests on a fundamental promise: if you work hard and contribute your labor, you can earn a living. This basic arrangement is the social contract that has organized human civilization for centuries. When you strip away the complexity, our entire economic and social structure is built around this exchange of labor for wages. We go to school, develop skills, and enter the workforce with the understanding that our efforts will be rewarded with the means to live and participate in society.
This wage-labor social contract forms the foundation of modern life, determining where we live, how we structure our time, and even how we define ourselves. Our legal systems, educational institutions, healthcare delivery, and social safety nets all presume this relationship between work and compensation.
But what happens when this fundamental arrangement becomes technologically impossible to fulfill? What occurs when businesses have every economic incentive to choose machines over humans, not because of greed, but because of rational economic decisions? This is the critical question we face as automation and AI continue their advance across the economy. The social contract that has organized our society is beginning to break down in ways that challenge our most basic assumptions.
Companies are increasingly choosing automation over human workers for straightforward economic reasons. When machines can perform tasks better, faster, cheaper, and safer than humans, businesses face competitive pressure to adopt these technologies. Labor costs typically represent 40-70% of business expenses depending on the industry – a massive portion that directly impacts pricing, competitiveness, and ultimately survival in the marketplace. When automation can reduce these costs while improving output, the economic calculus becomes clear.
The COVID-19 pandemic dramatically accelerated this trend. Facing lockdowns, social distancing requirements, and economic uncertainty, many businesses turned to automation as a solution. Warehouses deployed more robots to manage online order fulfillment. Restaurants installed self-service kiosks. Remote work technologies replaced in-person interactions. As James Martinez, a former warehouse supervisor in Ohio, told researchers, “In January 2020, we had 130 workers on the floor. By December, we had 60 workers and 12 new robots. Those jobs aren’t coming back.” What might have taken a decade of gradual adoption compressed into months of urgent implementation.
The data confirms this acceleration. Industrial robot installations increased by 31% year-over-year in 2021, with over 500,000 new robots deployed globally. Investment in AI startups reached record levels, with $66.8 billion invested in 2021 alone. Meanwhile, millions of workers who lost jobs during the pandemic found their positions permanently eliminated when the economy reopened.
This shift challenges the concept of the “right to work” in fundamental ways. Liberal democracies have long operated on the principle that citizens have the right to seek employment and earn a living. But what happens when that right becomes economically meaningless? When businesses have strong financial incentives to choose robots and AI over humans, the theoretical right to work becomes hollow. You can’t exercise a right to work when the work itself no longer exists in a form that humans can economically provide.
The breakdown of this social contract affects much more than employment statistics. Work provides identity, purpose, and social status. Throughout human history, one of the first questions we ask when meeting someone new is, “What do you do?” Our occupations form a core part of how we understand ourselves and how others perceive us.
When automation makes these identities economically obsolete, the psychological impact can be devastating. Studies of communities affected by large-scale job losses show increases in depression, substance abuse, domestic violence, and even suicide. “I used to be somebody in this town,” said Michael Brennan, a former manufacturing worker from Michigan. “Now I’m just another unemployed guy.”
Traditional policy responses have centered around job retraining programs. The theory is straightforward: as technology eliminates some jobs, workers can be retrained for new roles. This approach worked reasonably well during previous technological transitions. When agricultural automation reduced farm employment, workers moved to manufacturing. When manufacturing automated, many shifted to service roles and knowledge work.
But these solutions fail when there aren’t enough jobs for humans at any skill level. Retraining only works when positions are available for retrained workers. As automation and AI advance across all sectors simultaneously, the number of jobs requiring human input may decline faster than new positions are created. You can’t retrain everyone to be AI programmers or robot maintenance technicians—those fields won’t employ enough people to absorb all displaced workers.
This creates a profound challenge to our economic system. How do we structure an economy where an increasing percentage of the population cannot find work at living wages? These questions strike at the heart of our social and economic arrangements.
The situation becomes more urgent when we consider that our consumer economy depends on people having income to spend. Businesses need customers with purchasing power. If automation eliminates jobs without creating new mechanisms for people to earn income, the entire economic system faces a potential crisis. Companies may optimize their operations through automation only to discover they’ve eliminated their customer base.
Automation increases productivity and reduces costs—outcomes that should benefit society. Yet if these benefits accrue primarily to capital owners while eliminating the primary mechanism through which most people participate in the economy, we face a structural contradiction that the current system cannot easily resolve.
The resolution to this dilemma will require entirely new economic organizing principles. Some economists propose universal basic income as one potential solution. Others suggest digital dividends from data or automation taxes. Some envision new forms of public ownership of automated systems.
The question isn’t whether this transition will happen—it’s already underway. The real questions involve how we manage this shift, who benefits from increased productivity, and how we create new systems that provide both economic security and meaningful participation for everyone. When machines make everything but humans earn nothing, who’s left to buy the products? This contradiction creates a structural problem that challenges the viability of our entire economic model.
The Demand Paradox
We’ve arrived at perhaps the most perplexing economic challenge of the automation age: when machines make everything but humans earn nothing, who’s left to buy the products? This question highlights a fundamental contradiction that threatens the stability of our entire economic system.
The problem is straightforward but profound. Automation simultaneously increases productivity while eliminating the wages that fuel consumer spending. This creates what economists call the aggregate demand challenge – like sawing off the branch you’re sitting on while attempting to climb higher.
This paradox creates a potentially destructive economic cycle: Companies implement automation to reduce labor costs and increase productivity. Widespread job losses follow as human workers become economically unnecessary. Workers lose income, reducing their ability to purchase goods and services. Consumer spending drops, businesses see declining sales, and companies cut costs further with more automation. The cycle continues, potentially triggering economic contraction affecting even businesses that haven’t automated.
The retail sector demonstrates this paradox in action. Target has installed over 10,000 self-checkout systems nationwide, reducing cashier positions by 30% in some locations. Major retailers have aggressively implemented automated warehouses and AI-powered inventory management. An Amazon fulfillment center with advanced robotics processes the same volume of orders with 70% fewer workers than warehouses required a decade ago. Meanwhile, retail employment has declined by hundreds of thousands of jobs. These displaced workers can’t afford to shop at the very stores that automated them away – continuing the branch-sawing cycle.
What distinguishes this challenge from previous technological revolutions is both scope and speed. Previous shifts primarily replaced physical labor but created knowledge work. The industrial revolution moved people from farms to factories. Computerization moved people from factories to offices. But AI threatens both physical and knowledge work simultaneously, potentially eliminating job categories faster than new ones emerge. No clearly visible “next sector” could absorb displaced workers at comparable wages in similar numbers.
Economic models are capturing this contradictory dynamic. Projections show potential GDP growth alongside declining consumer purchasing power. This contradicts traditional economic thinking, where productivity growth translates to higher wages and increased spending. We’re entering a period where productivity increases through automation while wages stagnate or decline due to reduced demand for human labor.
Companies may successfully reduce costs through automation only to discover they’ve eliminated their customer base. A manufacturing company that automates 80% of its production line significantly reduces labor costs. But if similar automation occurs across industries simultaneously, who will have income to purchase those efficiently produced products?
This dynamic particularly threatens consumer-focused businesses. Companies selling discretionary products and services depend on consumers having disposable income beyond basics. As automation reduces employment and wages, discretionary spending typically gets cut first. These businesses face a double threat: pressure to automate their operations while watching their customer base erode as other industries automate.
From a macroeconomic perspective, this creates a potential underconsumption crisis. Production capacity may exceed consumers’ ability to purchase goods and services, leading to economic stagnation despite technological advancement. The economy could produce abundant goods while large population segments lack means to access them. This challenges the fundamental assumption of market economies: that supply and demand balance naturally when consumers have purchasing power derived from wages.
If automation advances across industries without alternative mechanisms for distributing purchasing power, we face a contradiction – unprecedented abundance alongside widespread economic precarity. This contradiction cannot sustain itself. Either purchasing power must be maintained through new distribution mechanisms, or production will eventually contract to match reduced consumer demand.
Traditional market economies presume most people participate as both producers (through labor) and consumers (through wages). When automation breaks this connection, the system lacks a natural balancing mechanism. This requires new models of value distribution beyond the wage-labor relationship.
Potential solutions include universal basic income, data dividends (where people receive payment for the use of their data), public ownership of automated systems, or new forms of labor unions capturing productivity gains from automation. Each approach addresses the core problem: maintaining consumer purchasing power when traditional employment becomes unavailable to large population segments.
Our economic system was designed for an era where human labor was essential to production. As we transition to an economy where machines produce with minimal human input, we need new organizing principles ensuring technological benefits are widely shared rather than concentrated in ways that undermine the system itself.
Resolving this paradox requires addressing ownership questions directly. Who owns the robots? Who benefits from AI productivity? How do we ensure technological advancement improves life for everyone rather than creating concentrated wealth alongside widespread insecurity?
The transition won’t be uniform across sectors. While automation will transform the entire economy, not all jobs will disappear simultaneously or at equal rates. Some human roles will persist longer, creating a complex transformation landscape. Understanding which jobs are likely to remain human-dominated provides crucial insights for individuals planning careers and for society preparing for structural economic change.
The Last Jobs Standing
Not all jobs will disappear at the same time. Some human roles will survive the automation wave longer than others—but not necessarily the ones conventional wisdom suggests. The pattern of which jobs persist longer offers important insights into how this transition will unfold.
What makes certain jobs resistant to automation isn’t necessarily complexity or education requirements. Instead, the most automation-resistant roles share a surprising characteristic: they exist in preference-driven employment niches—areas where humans specifically demand other humans, even when machines could technically do the job better or cheaper.
This insight challenges conventional wisdom about which jobs are “automation-proof.” For decades, career advisors suggested that highly skilled, complex, or creative jobs would be safest. The standard advice was to pursue education in medicine, law, or engineering to ensure job security. However, this perspective misses a crucial point: technical capability to automate a job doesn’t automatically mean society will accept that automation. The deciding factor is often whether humans specifically prefer human involvement, regardless of machine efficiency.
High-liability positions represent one of the strongest categories for continued human employment. These roles require personal accountability and responsibility for consequences. Judges making sentencing decisions, doctors determining treatment plans, or political leaders making policy choices remain in demand because society requires a human decision-maker who can be held personally accountable for outcomes.
This accountability requirement creates persistent demand for human involvement. If an automated system makes a medical diagnosis error, who faces the malpractice lawsuit? If an AI judge issues an unjust sentence, who bears moral responsibility? The need for someone to bear consequences—someone who can be fired, sued, or voted out of office—maintains human employment in these high-stakes positions.
Statutory positions form another category where human employment will persist longer. These roles have legal frameworks explicitly requiring human involvement. Professional certifications and licenses are legally structured around human qualifications and responsibility. Consider financial advisors – while algorithms can construct investment portfolios efficiently, regulatory frameworks still require human oversight. The advisor might use AI tools to analyze options but legally remains the responsible party who signs off on recommendations. These requirements typically lag technological capability by years, creating a buffer period for human employment.
The experience economy represents an area where human employment may persist despite automation capability. These roles make human interaction a central part of the value proposition. A skilled bartender doesn’t just mix ingredients according to recipes—they read the room, engage in conversation, remember patrons’ preferences, and create an atmosphere no machine can replicate. Similarly, musicians performing live or tour guides creating memorable experiences offer value beyond the functional outcome.
Neurological research suggests humans have innate social wiring that creates persistent demand for authentic human interaction. This explains why, even as recorded music quality improved dramatically, live concert attendance has grown rather than declined. The appeal of human interaction in these contexts is hardwired into our social nature, creating lasting employment opportunities in face-to-face roles.
“Meaning makers” represent another category likely to resist automation longer than technical capability might suggest. These people help others navigate existential questions through shared human experience. Therapists, spiritual advisors, coaches, and mentors provide value stemming from human connection and understanding of the human condition. Even as AI systems develop sophisticated capabilities to analyze psychological patterns, humans still seek connection with others who truly understand their experience.
Relationship and trust-based positions also maintain persistent human demand. Sales, diplomacy, and negotiation require building authentic connections and navigating complex social dynamics. A seasoned lawyer negotiating a settlement brings nuanced judgment, emotional intelligence, and the ability to build rapport that clients trust in ways they wouldn’t trust an algorithm. While AI can analyze negotiation strategies, the human ability to read subtle social cues and adapt to emotional contexts remains valuable.
The boundaries between human and automated work will likely shift gradually. Initially, automation will augment these roles rather than replace them entirely. A judge might use AI to analyze case precedents while making the final decision themselves. Over time, the human component may shrink to specific aspects where human presence adds unique value. Eventually, advances in emotional AI and robotic embodiment may challenge even these remaining domains.
Human employment will persist primarily in areas where humans specifically demand other humans for psychological, legal, or ethical reasons—even when machines could technically perform the tasks more efficiently. This preference-driven employment creates a buffer against automation that varies across cultures and changes over time.
This understanding helps individuals navigate career choices during this transition. Rather than simply pursuing technical skills, considering where human presence will remain valued offers insight into more durable employment prospects. Fields combining technical knowledge with human relationship skills may offer the strongest prospects as we move deeper into the automation age.
But this leads us to another crucial question: What about the classic argument that technology always creates more jobs than it destroys? Throughout industrial history, technological change has eliminated certain jobs while creating new ones—often in greater numbers. Will artificial intelligence and robotics follow this same pattern, or are we truly facing something different this time?
The Infinite Demand Fallacy
“Technology always creates more jobs than it destroys.” This reassuring belief has sustained us through centuries of technological change. When tractors replaced farm workers, manufacturing jobs emerged. When automation hit factories, service industry positions multiplied. When globalization shifted manufacturing overseas, knowledge economy roles expanded. This pattern has been so consistent that economists formalized it as the “lump of labor fallacy” – the understanding that technological progress creates entirely new categories of employment beyond what we could previously imagine.
The historical evidence appears compelling. The automobile eliminated horse-drawn carriage drivers but created millions of jobs in manufacturing, maintenance, sales, and eventually ride-sharing services. The personal computer transformed clerical work while spawning entirely new industries from software development to IT support. The internet created vast employment ecosystems around e-commerce, digital marketing, and content creation, despite disrupting traditional businesses. According to a 2020 World Economic Forum report, technological revolutions have consistently generated more jobs than they eliminated, with each revolution creating an average job growth of 10-15% over the corresponding displacement.
But what if artificial intelligence breaks this pattern?
Unlike previous technological revolutions that primarily replaced physical labor while creating demand for knowledge work, AI threatens both simultaneously. The steam engine replaced human muscle but created demand for human minds. The computer automated calculation but created demand for programmers and analysts. AI can potentially outperform humans across the entire spectrum of productive capabilities—physical, cognitive, and increasingly, emotional work.
This comprehensive automation capability distinguishes AI from earlier technological advancements. Farm mechanization pushed workers to factories, factory automation pushed workers to services, and early computerization pushed workers to knowledge roles. Each transition leveraged uniquely human capabilities. AI has the potential to close multiple doors simultaneously without necessarily opening new ones requiring human involvement. Research from Oxford University suggests that 47% of U.S. jobs face high risk of automation in the coming decades – a percentage far higher than during previous technological transitions.
The concept of infinite human demand provides an important counterargument. As technology reduces production costs through technological deflation, it liberates capital for new spending categories. When necessities become cheaper through automation, people develop new desires that didn’t previously exist. This explains employment growth throughout previous technological revolutions. As food production became more efficient, entertainment industries expanded. As manufacturing automation made goods cheaper, service industries grew. This pattern suggests human demands continuously evolve.
However, the crucial question isn’t whether new demands will emerge—they certainly will—but whether these demands will require human labor. While human demand may be limitless, there’s no economic law requiring these demands to be met by human workers rather than machines. If AI can satisfy both existing and emerging demands more effectively, the connection between market demands and human employment opportunities weakens significantly.
We’re seeing examples of new industries being born fully automated. Previous delivery innovations created millions of jobs for human drivers. The emerging autonomous delivery industry is designed to operate with minimal human intervention. AI content creation tools enable new media formats without traditional creative input. Virtual influencers, AI-generated music, and procedural video content represent markets producing value without proportional human employment.
The speed of AI advancement presents another challenge. Previous technological revolutions unfolded over decades, giving humans time to adapt. The steam engine didn’t displace all manual labor overnight. Computerization didn’t eliminate all clerical jobs in a single year. This gradual pace allowed education systems and labor markets to adjust. AI development is advancing exponentially, potentially outpacing human adaptation. McKinsey Global Institute estimates that AI could automate activities that currently require equivalent effort of 800 million full-time workers by 2030.
Language models have evolved rapidly from producing awkward text to generating human-quality content across many domains in just a few years. Similar advances are occurring in computer vision, robotic manipulation, and creative applications. This accelerating pace means that by the time humans identify safe havens from automation and retrain, those fields might become vulnerable to newer AI capabilities.
What happens when machines can fill new economic niches faster than humans? The traditional pattern of technological job creation depends on humans having time to identify opportunities and develop skills. If AI can rapidly adapt to economic demands without requiring years of education, the window for human workers in emerging fields may narrow significantly.
Some argue humans will always maintain advantages in creativity, emotional intelligence, and interpersonal skills. While these areas present greater challenges for automation, AI is advancing even in these domains. Creative AI generates emotionally resonant art and writing. Customer service AI detects emotional states and responds with appropriate empathy. These developments suggest even supposedly automation-resistant skills may eventually face AI competition.
The reassuring historical pattern of technology creating more jobs than it destroys reflected the specific limitations of previous technologies. AI potentially removes these limitations, creating the possibility of different outcomes from previous revolutions. This raises the critical question of who will control automated production systems in a world where machines handle most production. The distribution of benefits will depend not on who performs the work, but on who owns the machines – a question that may ultimately determine whether automation creates broadly shared prosperity or concentrated wealth alongside widespread economic displacement.
The Ownership Question
When machines do all the work, who owns the output? This isn’t just a philosophical question—it’s the central issue that will determine whether automation creates widespread prosperity or devastating inequality. As AI and robotics systems take over more economic functions, the critical question isn’t whether machines will outperform humans, but who will own these systems and control their output.
This ownership question strikes at the heart of how our future society will function. While we’ve spent considerable time examining how automation will transform work, we haven’t adequately addressed who will control these powerful production systems once they’ve replaced human labor. The structure of ownership will fundamentally shape how the benefits of technological productivity are distributed throughout society.
The stakes are enormous. Imagine automation as a vault containing unprecedented abundance – the question is who holds the keys. One path creates a world where technology provides necessities and luxuries to everyone with minimal human labor. Another path develops a system where production benefits flow primarily to those who own the automated systems, creating extreme inequality as most people lose both wages and economic relevance.
Currently, we face contrasting visions of how ownership might be structured in this automated future. The default path—without deliberate action—points toward increasing corporate concentration of automated systems. Large technology companies are acquiring AI startups and robotics firms, consolidating ownership of key automation technologies. This trend potentially leads to a future where a few corporations control the majority of productive capacity, creating unprecedented concentration of economic power.
At the opposite end lies collective ownership—the idea that automated production systems should be owned and controlled by broader segments of society. This concept updates the traditional Marxist idea of “seizing the means of production” for the age of automation. In the industrial era, this meant workers controlling factories. In a post-labor economy, it might mean collective ownership of the data centers, algorithms, and robots that produce economic value.
Collective ownership doesn’t necessarily mean state ownership or complete nationalization. While some advocate for national ownership of key AI infrastructure, others point to drawbacks of centralized control, including inefficiency, lack of innovation, and potential abuse of power. The question becomes what form that ownership should take to maximize both productivity and equitable distribution.
Several novel approaches could combine private initiative and collective benefit. Personal AI ownership represents one model, where individuals own AI systems that work on their behalf—similar to smartphone ownership today, but with AI that performs valuable economic tasks and generates income for its owner. This approach distributes ownership broadly while maintaining individual control.
Automation dividends offer another approach, sharing the benefits of automated production through direct payments to citizens. Drawing inspiration from the Alaska Permanent Fund, which distributes oil revenue to residents, a similar system might collect fees or taxes from automated production and distribute them as regular payments, ensuring everyone benefits from increased productivity even as traditional employment declines.
The “data as labor” model recognizes the value of data individuals generate, which trains and improves AI systems. Rather than treating data as freely collected, this approach would compensate people for their data contributions, creating a new form of economic value exchange. This framework acknowledges that modern AI depends on massive datasets created through human activity.
These approaches could work together. A functioning post-labor economy might incorporate elements of multiple ownership models, creating a hybrid system balancing efficiency with equitable distribution. What matters most is deliberately addressing the ownership question rather than allowing it to be answered by default through existing power structures.
Consumer preferences might also shape ownership structures. If consumers support companies with more equitable ownership models, market forces could drive the adoption of more distributed approaches. We already see this in sectors where consumers choose companies based on environmental or social practices.
The decisions about ownership will shape society for generations. If automated systems remain primarily owned by corporations and shareholders, productivity gains will flow mainly to capital owners. If broader ownership models emerge, the benefits could be widely distributed, creating a more equitable society.
History offers relevant lessons. The Industrial Revolution initially led to terrible working conditions and inequality before broader social reforms distributed its benefits more widely. The digital revolution has similarly concentrated wealth among technology companies and investors. With AI and robotics, we have an opportunity to proactively shape the distribution of benefits.
In a highly automated economy, traditional policy tools lose effectiveness. Minimum wage laws matter less when fewer jobs exist. Labor protections become less relevant when machines do most work. Education and training can’t solve structural unemployment if machines can perform most tasks more efficiently. Ownership structures become the primary determinant of economic outcomes.
For businesses, this revolution creates both opportunities and threats. Companies seek efficiency through automation, but collectively face a paradox: if automation eliminates too many jobs without creating new income mechanisms, who will buy their products? The individually rational decision (automate to reduce costs) leads to a collectively irrational outcome (eliminate consumer purchasing power).
This paradox highlights why ownership structures matter so much. If automation benefits flow primarily to capital owners, aggregate demand may collapse as wages disappear. If benefits are more widely distributed through alternative ownership models, consumer purchasing power could be maintained even as traditional employment declines.
The ownership of automated systems represents the central economic question that will determine whether post-labor economics creates prosperity or poverty. Everything else—job displacement, skill development, social safety nets—flows from this fundamental question.
This transitions us to examine the business perspective in more detail. For companies, the mathematical reality is straightforward but potentially problematic: the ideal number of employees is approaching zero. What happens when this becomes possible across entire industries, and what paradoxes does it create for businesses themselves?
The Zero-Employee Endgame
From a pure business perspective, the math around employment becomes increasingly simple: the ideal number of employees approaches zero. This isn’t a statement about corporate values or ethics—it’s the cold reality of balance sheet mathematics. Human employees represent the most expensive and complex asset any company manages. When businesses look at their financial statements, labor costs typically account for a significant portion of total expenses across industries. This makes workforce reduction through automation an irresistible target for companies seeking to improve their financial performance.
The complexity of managing human resources extends far beyond wages. Companies must navigate health insurance, retirement benefits, paid time off, workers’ compensation, unemployment insurance, training costs, management overhead, workplace facilities, and compliance with countless labor regulations. A factory building is relatively simple—four walls and machinery. A human workforce requires an entire ecosystem of support systems, each adding layers of cost and complexity.
This creates what we might call the automation imperative: as AI and robotics become better, faster, cheaper, and safer than human workers, companies operating with rational self-interest will inevitably choose to automate. This is about survival in competitive markets. When your competitors reduce their costs through automation, you face a brutal choice: follow suit or lose market share as your prices become uncompetitive.
Consider what happened with automated checkout systems in retail. When major retailers first implemented self-checkout, they gained a small cost advantage. As the technology improved and customer acceptance grew, these systems became standard. Retailers who failed to adopt them found themselves at a disadvantage. The same pattern is now repeating with more advanced automation across sectors—companies that resist automation risk falling behind competitors who embrace it.
The manufacturing sector provides the clearest example of progression toward zero-employee operations. “Lights-out manufacturing” refers to fully automated factories that operate without human presence—literally with the lights turned off. These facilities run 24/7 with minimal human oversight, producing goods with consistent quality and lower operating costs. One Japanese electronics manufacturer converted its facility to near-complete automation, reducing defects by 70% while cutting production costs by 30%. When asked about the difficult decision to lay off long-term employees, the CEO remarked, “We either automated or closed entirely. At least this way, some management positions remained.”
Service industries are following a similar trajectory, though at a different pace. Customer service AI now handles increasingly complex interactions. Financial services automate everything from loan approvals to investment management. Creative services see growing automation through AI-generated content, designs, and media. Each wave of automation follows the same pattern: initial adoption by industry leaders creates competitive pressure that eventually makes automation the standard approach.
What makes this transition particularly challenging is the existential paradox companies face: eliminating their largest expense while somehow maintaining customer purchasing power. This creates the automation dilemma. If a single company automates and reduces its workforce, it gains a competitive advantage. If all companies do the same, they collectively undermine the consumer purchasing power necessary for their products to find buyers.
Corporate survival and consumer demand are inextricably linked. Both depend on maintaining customers with sufficient disposable income. The elimination of employees through automation leads to a decline in consumer purchasing power, which then collapses demand for products, creating economic contraction. Companies, by automating and reducing their workforce, erode the very foundation of their customer base.
Different industries approach this zero-employee model at varying speeds. Manufacturing leads with highly automated production lines. Retail follows with increasingly automated stores. Professional services transform through AI systems that analyze documents, generate content, and process information. Even healthcare, education, and creative fields see growing automation of tasks once considered uniquely human.
The question businesses increasingly face is stark. How will they survive when their customers no longer have income from employment? Without wages flowing to potential customers, who will buy the products coming off efficient automated production lines? This creates a fundamental contradiction in our economic system—companies optimize for minimal human involvement while depending on humans having disposable income to purchase their products.
Some businesses recognize this contradiction and explore alternative approaches. These include supporting universal basic income proposals, developing hybrid human-machine workforces, or creating new business models that distribute value differently. Others focus simply on being the most efficient competitor, assuming broader economic questions will resolve themselves or that government policies will eventually address systemic challenges.
From a business mathematics perspective, zero employees increasingly appears optimal. Every human worker replaced by automation represents a permanent reduction in operating costs, management complexity, and liability exposure. Companies that optimize for this reality gain advantages over competitors who move more slowly. Yet this optimization, when multiplied across the economy, creates structural contradictions that threaten the foundation of our market system.
This transition from human labor to automated production requires us to completely rethink how people obtain economic agency in society. For centuries, the wage-labor relationship has been the primary mechanism through which most people participate in the economy. If that mechanism becomes increasingly unavailable as businesses optimize toward zero employees, we need new frameworks for ensuring people can participate meaningfully in economic activity.
The zero-employee endgame is a fundamental transformation of our economic system that challenges basic assumptions about how society functions. Companies will continue to pursue automation because the business case is compelling, but the collective impact of these individual decisions requires us to develop entirely new mechanisms for distributing purchasing power and economic rights in a world where traditional employment may no longer serve as the primary connection between production and consumption.
Reinventing Economic Agency
If a future without jobs sounds scary, that’s because it challenges the very foundation of how we participate in society. Throughout history, your ability to influence your financial situation—what economists call economic agency—has rested on three critical pillars: the wages you earn, the property you own, and your democratic rights as a citizen. These three elements work together to give you economic power. But what happens when one of these pillars—specifically, your ability to earn wages—begins to crumble?
Today, most people derive their economic power primarily through employment. You work at a job, earn a paycheck, and use that money to participate in the economy as a consumer. This wage-labor relationship provides the primary mechanism through which most people acquire property and build wealth. Your paycheck allows you to buy a home, invest in stocks, or start a business.
Automation directly undermines this traditional pathway. Consider Sarah, a paralegal who spent fifteen years building expertise in document review. When her firm implemented AI software that could analyze contracts in seconds, her hours were cut by 70%. Despite her skills, she couldn’t maintain her mortgage payments. Her economic agency—her ability to participate meaningfully in the economy—dramatically diminished despite her willingness to work.
When machines perform most productive tasks more efficiently than humans, the wage-labor social contract begins to break down. The mechanisms through which ordinary people have traditionally built wealth and security disappear.
This creates a challenging situation. Our consumer-based market systems depend on people having money to spend. But if automation boosts productivity while simultaneously reducing wages flowing to workers, who will buy all these efficiently produced goods and services? This contradiction threatens the stability of our entire economic system. Current consumer-based market systems may falter when automation increases productivity but traditional employment-based purchasing power disappears.
To address this challenge, we need alternative models for economic participation not exclusively dependent on employment. Universal basic income represents a straightforward solution—providing regular cash payments to all citizens regardless of work status. This approach directly addresses the income side of the equation, ensuring people can participate as consumers even when traditional jobs are scarce.
Data dividends offer another approach. The AI systems automating our economy depend on massive amounts of data—much of it generated by ordinary people. Think of it like oil royalties: if companies profit from your data, you deserve compensation. This creates a new form of economic value exchange not dependent on traditional employment.
Algorithmic royalties extend this concept. As AI systems generate value by creating content or performing services, they could direct a portion of that value back to contributors. Imagine receiving small payments whenever an AI system trained on public conversations produces commercially valuable output—similar to how musicians receive royalties when their songs are played.
The digital commons represents another approach. This involves creating shared resources anyone can access—similar to how open-source software functions today. In this model, certain automated production systems would be publicly owned, with benefits distributed broadly rather than captured privately, like a community garden where everyone contributes and everyone harvests.
As automation transforms the economy, our concept of property rights may need to evolve. Rights to computational resources—the processing power and algorithms driving automation—could become as valuable as physical property. Similarly, data rights might evolve into a form of property individuals can control or sell.
Democratic economic rights could also expand beyond traditional voting. We might develop new mechanisms for direct economic participation—like citizen juries that help allocate community resources or participatory budgeting systems. These approaches could give people meaningful economic agency even without traditional employment.
Banking systems will play a critical role in any post-labor economic system. As traditional wages decline, banking systems might evolve to maintain consumer purchasing power through new mechanisms. Banks are positioned as critical architects due to their role as economic infrastructure, providing essential services that enable transactions and overall economic stability.
Several early experiments in post-labor economic systems are already underway. Finland, Kenya, and Canada have tested various forms of basic income. Estonia has pioneered digital citizenship that provides economic rights beyond traditional national boundaries. These experiments provide valuable insights into how alternative economic models might function at scale.
The transformation to post-labor economics won’t happen overnight. Government intervention will likely play an important role in managing this transition. The government’s role involves balancing competing needs to provide security, stability, and mediation among economic stakeholders. However, cultural preferences against significant state economic involvement create barriers to implementing the solutions required by automation challenges.
This reinvention of economic agency addresses the fundamental contradiction businesses face in the automation age. From a business perspective, the optimal number of employees is approaching zero—but this creates a paradox. As companies automate to reduce costs, they collectively undermine consumer purchasing power. New models of economic participation could resolve this contradiction by maintaining purchasing power even as traditional employment declines.
Society must develop new economic organizing principles that ensure people have economic agency without traditional employment. This represents a fundamental reimagining of how people participate meaningfully in society.
All of this leads us to ask—what does a post-labor future actually look like for individuals? How can you navigate this transition in your own life, and what strategies might help you thrive in this new economic reality?
Navigating the Transition
The transition to a post-labor economy isn’t some far-off scenario—it’s happening right now. What makes this shift different from previous technological revolutions is the pace and breadth of change. While the Industrial Revolution unfolded over generations, the AI revolution is compressing dramatic economic transformation into years or even months. This creates both urgent challenges and unique opportunities for anyone concerned about their economic future.
We’re experiencing an accelerating process through waves of automation. Manufacturing jobs were the first affected, followed by predictable service jobs, and now AI systems are challenging knowledge workers and professionals. Each successive wave moves faster, putting careers once considered technological-proof at risk within 5-10 years.
Consider Maria, a paralegal with 15 years of experience who discovered that 70% of her document review work could be performed by AI. Rather than resisting, she pivoted to become an AI-legal liaison, training systems and focusing on client relationships—doubling her income while working fewer hours.
Planning becomes challenging with uncertain automation timelines. Expert predictions range from rapid displacement within a decade to gradual transitions over 20-30 years. This timing variability directly impacts your decisions about education, career changes, and financial planning. The transformation differs across industries and regions, making personalized strategies essential.
To assess your career’s automation risk, examine your role through our four labor categories: strength, dexterity, cognition, and empathy. Roles heavily dependent on the first three categories face greater risk as technology rapidly advances in these domains. Information processing, procedural work, data analysis, and physical tasks have higher automation potential regardless of your industry or credentials.
Next, evaluate whether you work in a preference-driven employment niche—areas where humans specifically demand other humans. High-liability positions requiring accountability, statutory roles needing human licensure, experience economy jobs, and relationship-based positions tend to resist automation longer, even when technical capabilities exist.
Additionally, consider whether your job involves tasks challenging for AI: handling novel situations, navigating ambiguity, understanding cultural contexts, or building genuine emotional connections. These areas provide some protection against immediate displacement.
Several skill categories complement rather than compete with advancing technology. First, develop capabilities enhancing human-to-human interaction: advanced communication, negotiation, persuasion, and emotional intelligence. James, a former data analyst, transitioned to client success management when his technical work became automated, leveraging his interpersonal skills to become indispensable.
Second, learn to work alongside AI systems by understanding how to direct and interpret AI outputs, recognizing their limitations, and integrating them into workflows. The human+AI combination typically outperforms either working independently.
Third, cultivate cross-disciplinary knowledge connecting domains creatively. While AI excels within established parameters, it struggles with novel combinations across fields. Your ability to synthesize knowledge from multiple disciplines creates value that automated systems find difficult to replicate.
To position yourself in preference-driven employment niches, seek roles where accountability, judgment, or meaningful connection form the central value proposition. Healthcare providers combining technical knowledge with empathy, educators adapting to individual learning needs, creative professionals understanding cultural nuance, and advisors building trust operate in these protected spaces.
Consider roles involving high-stakes personal decisions where people want human judgment. Legal, financial, healthcare, and educational decisions with significant personal impact maintain human involvement longer, even when technical aspects could be automated.
For alternative income sources beyond traditional employment, explore generating revenue from assets rather than labor—intellectual property, investments, or digital products that create income without continuous direct effort. The CreatorPass platform enables artists to earn royalties when AI systems use their style, creating passive income streams while they focus on commission work.
Consider participating in emerging value distribution mechanisms: data dividends from your information, algorithmic royalties from AI systems trained on collective data, or digital commons where value is shared among contributors. Estonia’s digital residency program demonstrates how citizenship-based data rights can translate to economic benefits.
Develop multiple income streams across different risk categories. Like diversified investment portfolios, your “career portfolio” should include various value generation methods—traditional employment, freelance work, passive income, and alternative economic models—creating resilience against disruption.
Education must evolve for a post-labor world. Current systems prepare people for an employment-centered economy that may not exist when today’s students enter the workforce. Focus instead on adaptability over specific vocational training, emphasizing broadly applicable capabilities like critical thinking, creative problem-solving, and social intelligence.
Educational approaches developing uniquely human capabilities provide greater value as automation advances: social-emotional learning, ethical reasoning, cultural understanding, and creative expression. Lambda School’s income-share agreement model demonstrates education adapting to this new reality by aligning incentives with student outcomes.
Continuous, self-directed learning becomes essential in rapidly changing environments. The ability to identify knowledge gaps, find resources, and integrate new skills becomes core to navigating economic transitions.
Your preparation now will significantly influence whether this transition becomes devastating or liberating personally. Those who recognize the shift early, develop complementary skills, position themselves in preference-driven niches, and explore alternative income models will navigate more successfully than those clinging to traditional employment until it disappears.
These individual strategies connect to a broader societal transformation. Our collective choices about structuring a post-labor economy will determine whether automation creates broadly shared prosperity or concentrated wealth alongside widespread insecurity.
Conclusion
We stand at a turning point in human history. The post-labor economy represents a complete reimagining of civilization itself. For centuries, work has defined our lives, our identities, and our social structures. As automation transforms this fundamental relationship, we face both extraordinary challenges and unprecedented opportunities.
Imagine a world where humans are freed from labor constraints, able to pursue meaning and purpose beyond employment. Like a bird released from its cage, humanity could soar to new heights of creativity and fulfillment.
The decisions we make now about ownership, distribution, and economic agency will determine our collective future. Will we create shared abundance or concentrated power? The choice is ours.