Despite high expectations, AI isn’t skyrocketing productivity because many organizations face hurdles like integration delays, data quality issues, and workforce resistance. AI tools often still excel in simple tasks, and implementing them takes time and resources. Plus, cultural and ethical concerns slow down adoption, and real-world results lag behind optimistic forecasts. If you want to understand why those promised gains haven’t fully materialized yet, there’s more to explore below.
Key Takeaways
- Overestimated AI productivity gains are hindered by implementation delays, data quality issues, and integration challenges.
- AI’s current capabilities mainly improve simple tasks, limiting widespread impact on overall productivity.
- Organizational resistance, workforce fears, and ethical concerns slow adoption and effective utilization.
- Standardization, compatibility, and infrastructural hurdles delay seamless AI integration into existing systems.
- Real-world barriers cause actual productivity increases to fall short of optimistic projections and expectations.
The Promised Productivity Gains From AI
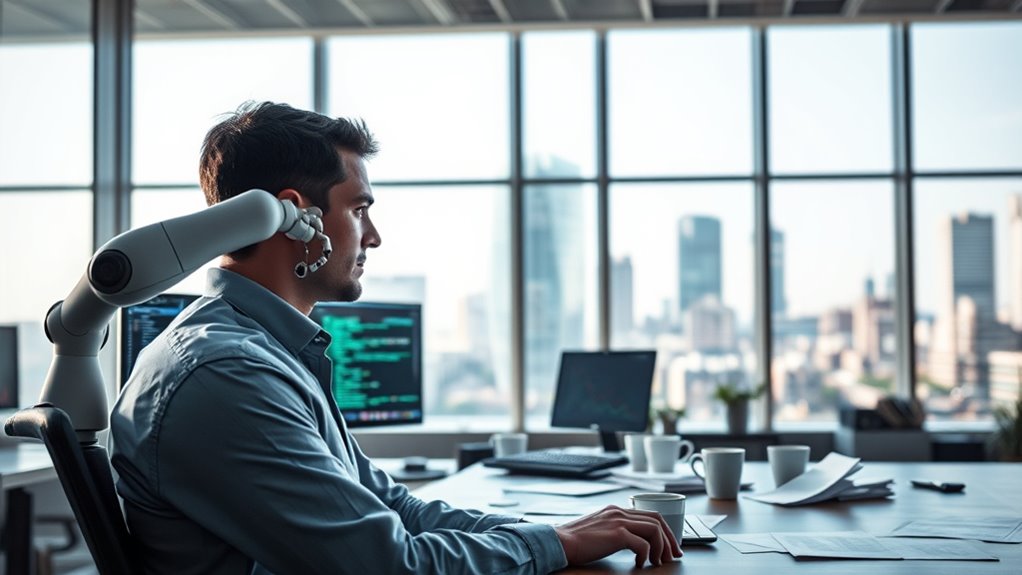
Have you ever wondered how much AI can truly boost productivity? According to McKinsey, AI could add up to $4.4 trillion to global productivity. PwC predicts that AI will improve employee efficiency by 40% by 2035. Over half of businesses expect AI to considerably enhance their productivity. Generative AI, in particular, is expected to automate tasks and foster innovation, helping companies save time and resources. As AI adoption accelerates—rising from 55% to 78% in just one year—you can see its growing influence across industries like manufacturing and banking. AI’s role in automating repetitive work and aiding decision-making is already transforming workflows. AI investment is increasing rapidly, with 92% of companies planning to expand their AI efforts in the next three years, these projections highlight AI’s massive potential to generate economic value and reshape how we work, promising remarkable gains in productivity. Additionally, the integration of natural language processing tools is making workflows more efficient by enabling faster data analysis and communication. Moreover, the adoption rate of AI technologies indicates a significant shift in corporate strategies toward automation and innovation, which could further accelerate productivity improvements over time. Furthermore, understanding the impact of AI on jobs can help organizations better prepare for ongoing changes in the workplace. Recognizing the importance of home decor innovations can also inspire companies to develop AI-driven tools that enhance interior design and organization solutions, creating more personalized and efficient spaces. A better understanding of technological integration can further facilitate smoother transitions and maximize benefits.
Discrepancies Between Expectations and Reality
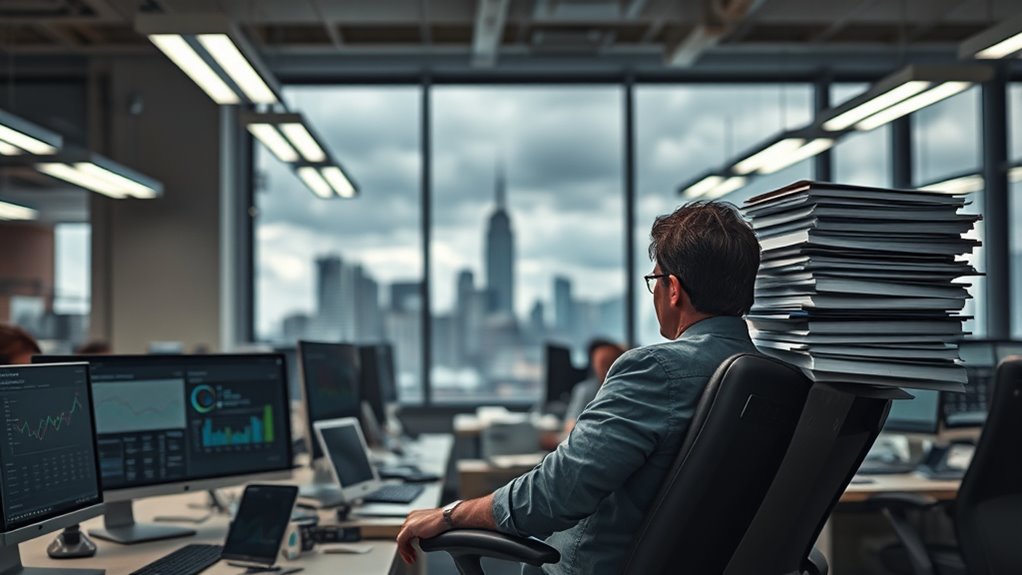
You might expect AI to instantly boost productivity, but the reality shows many organizations are still catching up. Adoption rates remain low, and challenges like data security and skills gaps slow progress. As a result, the productivity gains often fall short of initial expectations. Additionally, the current capabilities of AI agents are limited—they excel in simple tasks but still require significant human oversight for more complex workflows capabilities are limited. Furthermore, ongoing concerns about AI vulnerabilities and biases hinder widespread trust and adoption, which further dampens potential productivity improvements. Even in areas like smart home device integration, where technology has advanced, seamless compatibility remains a challenge for many users. Improving integration across diverse systems continues to be a barrier to realizing AI’s full potential in everyday applications. Moreover, the slow pace of technological standardization complicates efforts to achieve widespread interoperability, further limiting productivity gains.
Overestimated Productivity Gains
While many believe AI will revolutionize productivity overnight, the reality is often less dramatic. Expectations for massive gains are overestimated because implementation challenges slow progress. McKinsey estimates AI could add $4.4 trillion in productivity, but realizing this depends on overcoming complex hurdles like data quality and system integration. Users report saving about 5.4% of work hours, yet this only results in a 1.1% boost in overall productivity. Despite the optimism—64% of businesses believe AI will improve productivity—actual gains remain modest. Long-term projections suggest AI could increase productivity by up to 40%, but this remains a future goal. For now, the perceived benefits often fall short, as real-world barriers limit the immediate impact AI can deliver. Additionally, mindfulness techniques can help workers manage stress and maintain focus amidst these challenges. Developing a focused mindset can further enhance the effective use of AI tools in daily work routines. Moreover, adoption barriers such as organizational resistance and skill gaps hinder widespread implementation and impact. Recognizing and addressing personality traits that influence workplace dynamics may also facilitate smoother integration of AI-driven processes. Furthermore, embracing a holistic SEO approach ensures that organizational efforts align with broader productivity and content strategies, maximizing AI’s potential benefits.
Adoption Challenges Persist
Despite high expectations, many organizations still face significant hurdles when adopting AI, revealing a gap between anticipated benefits and actual implementation. Data quality and bias remain major concerns; nearly half of organizations worry about accuracy and fairness, which hampers trust and decision-making. Limited proprietary data and increased restrictions reduce model diversity and effectiveness. Privacy and security issues are also significant barriers, with organizations struggling to handle sensitive data while complying with regulations. Additionally, integrating AI into existing IT systems proves costly and complex, causing scalability issues. Without clear ROI or sufficient in-house expertise, many organizations hesitate to fully commit. These challenges slow adoption, preventing AI from delivering the productivity gains many expected. Overcoming these hurdles requires addressing data, security, infrastructure, and talent gaps. Moreover, the AI in Education subfield highlights how ongoing technological advancements and ethical considerations influence the pace of AI adoption across sectors. For example, the need for specialized expertise in deploying AI solutions is often underestimated, further delaying widespread implementation. Developing a comprehensive understanding of the technological and ethical landscape is essential for organizations to navigate the complex environment of AI integration effectively, especially as the role of creativity in innovation evolves alongside AI capabilities. Recognizing the importance of GMC tuning techniques can also aid in optimizing AI models for specific applications, ultimately helping to bridge the productivity gap.
Implementation Lag Hinders Impact
Implementing AI often takes longer to translate into measurable productivity gains than organizations initially expect. You might see rapid improvements in early projects, but widespread, lasting benefits usually lag. Several factors contribute to this delay:
- You need to train employees and integrate AI tools into existing workflows, which takes time and effort. Additionally, organizational change management is essential for smooth adoption.
- Organizations often require process redesigns and change management before realizing full benefits.
- The broader economy shows slower growth because many firms are still in early adoption stages or facing sector-specific hurdles.
- Technological integration can be complex, requiring updates to legacy systems and ensuring compatibility across various platforms.
These delays create a gap between initial expectations and actual results. As a result, productivity boosts appear fragmented, uneven, and sometimes invisible in macroeconomic data, even though individual firms are making progress.
Challenges in Fully Integrating AI Solutions
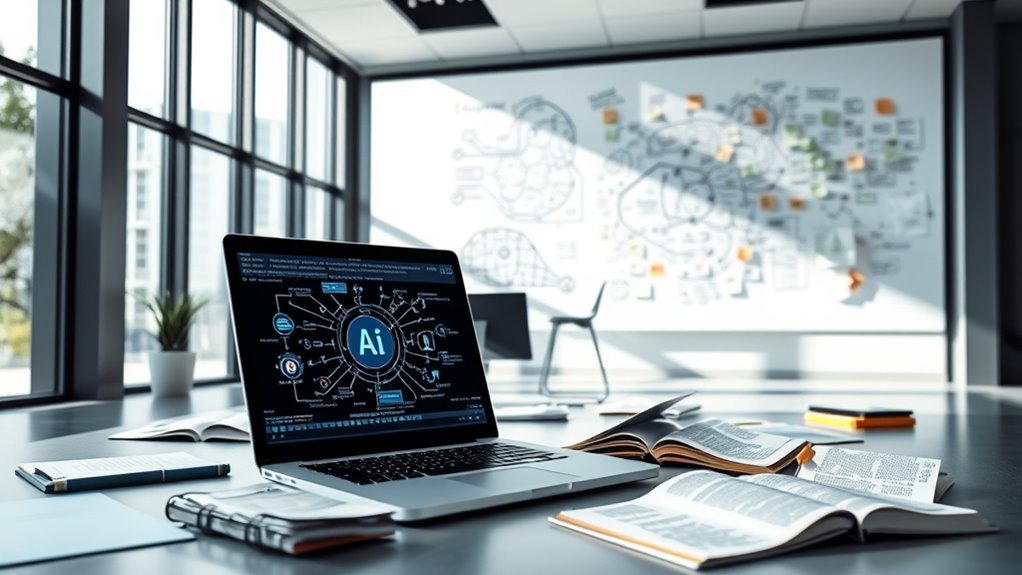
Integrating AI solutions into existing systems presents numerous challenges that organizations must navigate carefully. Technical complexity is a major hurdle, with over 90% of organizations struggling to connect AI with legacy systems. Ensuring data is clean and ready for integration often gets overlooked, yet it’s critical for success. Compatibility issues between AI and current IT infrastructure can cause delays, while a lack of technical expertise hampers smooth implementation. Scalability remains a significant concern, with 74% of companies finding it hard to extract sustained value. Additionally, data governance and cybersecurity concerns complicate integration efforts, as organizations must safeguard privacy and meet regulatory standards. Data management is essential for effective AI deployment, yet many organizations lack the necessary infrastructure to support it. All these factors create a complex landscape that slows down full AI adoption and limits its productivity impact. Organizational readiness also plays a crucial role, as many companies lack the strategic frameworks needed to effectively scale AI initiatives. Furthermore, the importance of aligning AI efforts with vibrational energy and organizational culture can influence the overall success of AI integration.
The Role of Workforce Resistance and Adoption Barriers
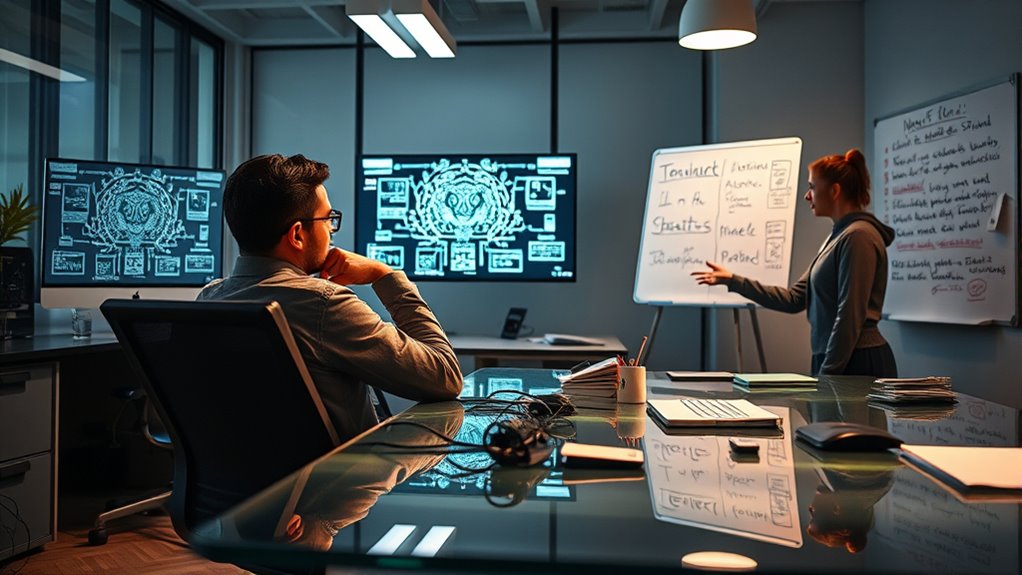
You might find that resistance to change and fear of losing jobs slow down AI adoption in your organization. Bridging the skill gap is essential, as many workers lack the training needed to work effectively with AI. Building trust in the technology is also pivotal for overcoming skepticism and ensuring smooth integration. Significant workforce displacement, estimated at 300 million jobs globally, underscores the importance of proactive adaptation strategies.
Resistance to Change
Workforce resistance remains a significant obstacle to AI adoption, fueled by uncertainty, fear, and cultural barriers. You might worry about when and how to use AI at work, feeling unsure of its purpose or acceptance. Many employees fear that using AI could make them look lazy or replaceable, leading to hesitation. Imagine these barriers as:
- Confusion over AI tools, like trying to navigate a complex maze without a map.
- Fear of being judged or perceived as incompetent by colleagues or managers.
- Cultural norms that discourage open discussions about AI use, creating walls instead of bridges.
These factors create a climate where resistance persists, slowing down progress. Overcoming resistance requires addressing these emotional and cultural hurdles, so AI can be embraced as a tool for growth rather than a threat. Research shows that addressing these concerns with clear communication and supportive onboarding can significantly reduce resistance.
Skill Gap Challenges
Resistance to change often stems from uncertainty and fear about how AI will impact jobs and daily tasks. You might worry about feeling unprepared or falling behind if you don’t learn new skills. Many workers lack proper training or digital literacy, making it harder to see AI as a helpful tool rather than a threat. Without clear guidelines, employees may feel unsure about how to use AI responsibly, leading to hesitation or shadow AI practices. Cost barriers also prevent small businesses and traditional trades from adopting AI effectively. Upskilling and aligning training with workforce education levels are essential, but companies often struggle with these efforts. Overcoming these skill gaps requires open communication, leadership support, and strategies that address employees’ fears and uncertainties to foster wider acceptance.
Trust in Technology
Why does trust in AI technology remain a significant hurdle to widespread adoption? Many workers feel uncertain about relying on AI, fearing they’ll be judged negatively or seen as incompetent. This discomfort leads to resistance, slowing down progress. Imagine these scenarios:
- You hesitate to use AI tools, worried your boss might think you’re lazy or unqualified.
- Employees avoid discussing AI use openly, fearing negative perceptions.
- Without clear guidance, workers feel overwhelmed and unsure when or how to incorporate AI into their tasks.
These barriers are compounded by a lack of organizational support—only 44% feel moderate to fully supported—and fears of job loss or technophobia. Overall AI adoption among desk workers worldwide is stalling. Until trust improves through better communication and training, AI adoption will stay sluggish, limiting productivity gains.
Limitations of Current AI Technologies
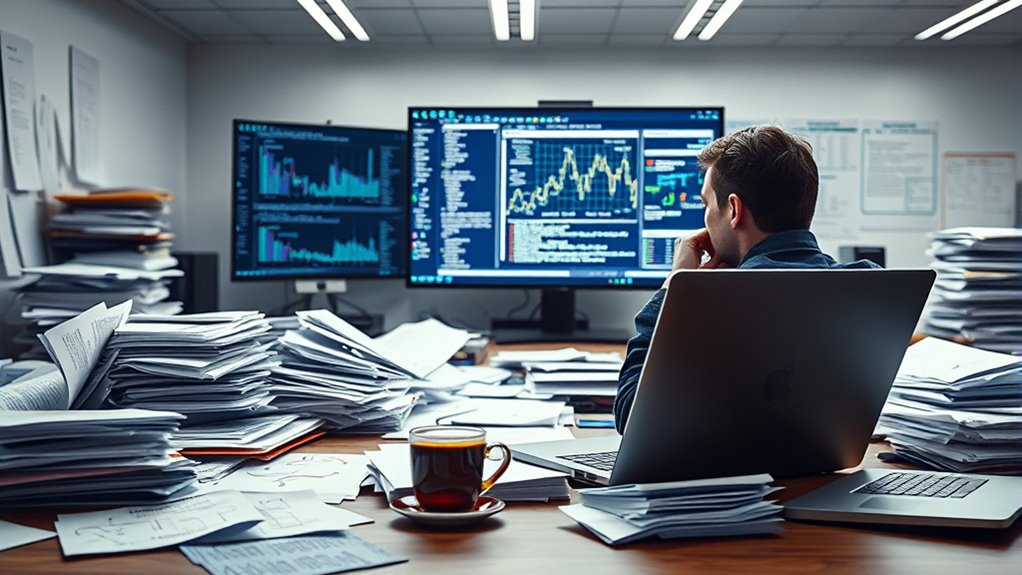
Current AI technologies face significant limitations that hinder their widespread adoption and effectiveness. One major issue is technical integration; many AI systems struggle with compatibility when working alongside legacy infrastructure. Scalability is another challenge—expanding AI for real-time tasks requires huge computational resources and careful optimization. Hardware demands are high, often needing upgrades to handle larger models. Cloud environments add bottlenecks, such as high memory use and data transfer delays. These technical barriers affect deployment and performance.
Limitation | Impact |
---|---|
Legacy System Compatibility | Difficult integration slows adoption |
Scalability Challenges | Limits real-time application efficiency |
Hardware Requirements | Increases infrastructure costs |
Cloud Bottlenecks | Causes delays and reduces system responsiveness |
Optimization Needs | Essential for effective operation in critical tasks |
Data Quality and Ethical Concerns Impacting Effectiveness
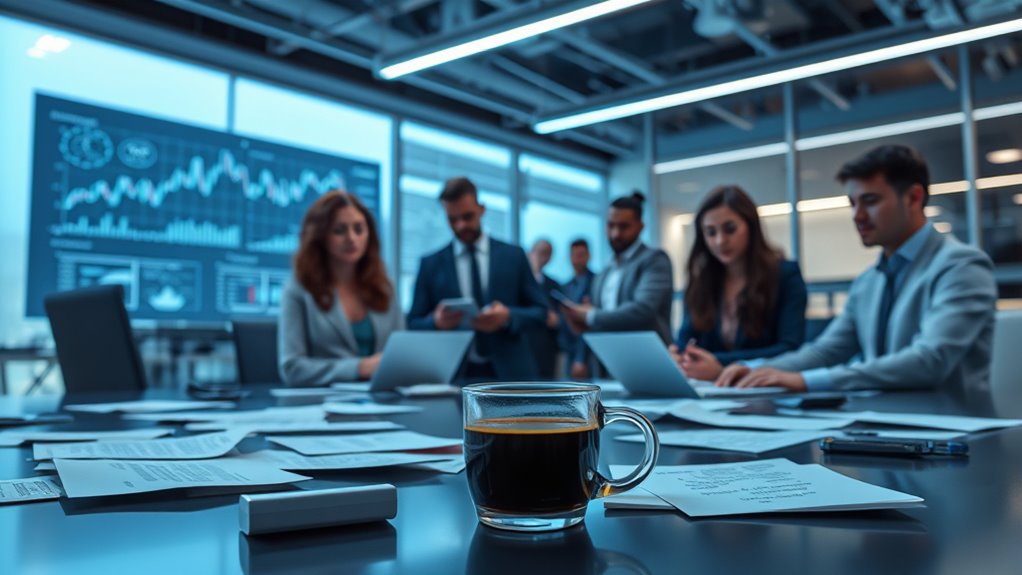
While technical limitations hinder AI deployment, data quality and ethical concerns directly affect how effectively AI systems perform and gain trust. Poor data accuracy can produce biased models and faulty predictions, while misconceptions about data quantity—thinking more always equals better—can cause overfitting and inefficiency. Biases in training data can lead AI to make unethical decisions, especially in sensitive areas like hiring or criminal justice. To visualize this, imagine:
- AI making unfair choices because of biased training data.
- An overfitted model that performs poorly on real-world data.
- Lack of transparency eroding user trust.
Addressing these issues requires improving data representation, balancing data quality and quantity, and establishing ethical standards. Only then can AI access its true productivity potential without compromising fairness or accuracy.
The Lag Between AI Advancements and Implementation
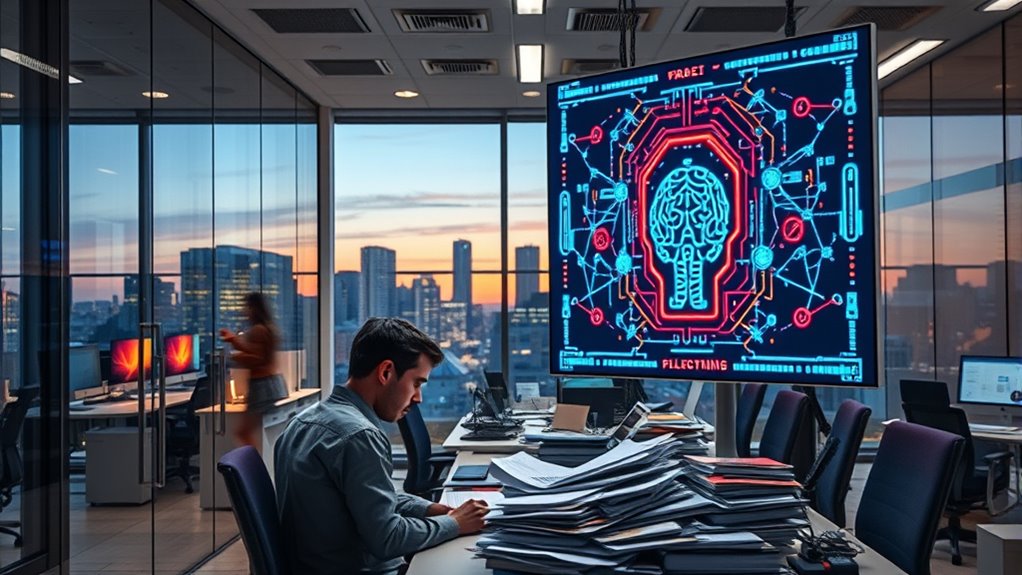
You may notice that AI advancements often take time to be fully adopted across industries. This delay is driven by integration challenges, regulatory hurdles, and ethical concerns that slow down implementation. Overcoming these barriers requires coordinated efforts and strategic planning to realize AI’s productivity benefits.
Adoption Delays and Hesitations
Despite rapid advancements in AI technology, organizations often struggle to implement these innovations promptly, leading to significant delays. You may see resistance from employees who fear job loss or feel uncomfortable with new workflows. They might:
- Cling to familiar routines, resisting change that disrupts their routines.
- Lack trust in AI’s decision-making, hesitating to rely on new tools.
- Feel unprepared due to insufficient training, leaving skills gaps unaddressed.
These fears and gaps slow adoption, as leadership may lack clear AI strategies or fail to secure the necessary resources. Budget constraints and organizational silos further hinder progress. The combination of cultural resistance and strategic misalignment creates a significant lag between AI advancements and their real-world application.
Integration Complexity Challenges
Integrating advanced AI systems into existing organizational infrastructure often presents significant technical challenges. Many organizations struggle with compatibility issues, requiring costly upgrades to IT systems. You may find it hard to locate skilled personnel who can effectively implement and manage AI, leading to delays. Poor data quality, including biases and gaps, can limit AI’s accuracy and usefulness. The high costs and resource demands of AI integration make justifying investments difficult, especially when benefits aren’t immediately clear. Scalability and adaptability pose additional hurdles, as AI solutions must evolve with your business needs. These complexities slow down deployment, increase risks, and often require organizational changes, making it even harder to realize AI’s productivity potential. Overcoming these barriers is essential before AI can truly transform your operations.
Regulatory and Ethical Barriers
The rapid pace of AI innovation has far outstripped the development of all-encompassing regulatory standards worldwide, creating significant uncertainties for businesses. You face a landscape where:
- Differing regulations across countries mean steering through complex legal terrain and risking non-compliance.
- Ambiguous rules around AI accountability slow your willingness to deploy new solutions broadly.
- Strict privacy laws, like GDPR, limit access to vital training data, hindering AI’s potential.
These barriers increase compliance costs and delay implementation. Ethical concerns—such as bias, transparency, and societal impact—further complicate adoption. Fear of job displacement and opacity in AI decision-making reduce trust. Meanwhile, slow legislative progress struggles to keep pace with technological advancements, leaving you in a state of regulatory limbo.
Industry-Specific Factors Influencing Productivity Outcomes
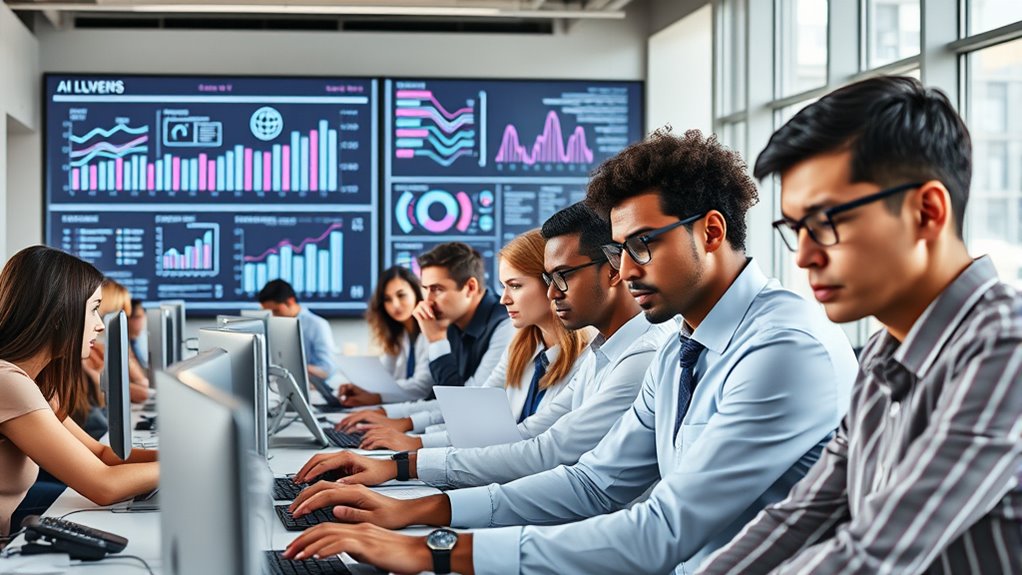
Industry-specific factors play a pivotal role in shaping productivity outcomes by influencing how effectively businesses adopt and utilize AI and other technological advancements. Your industry’s structure matters; tech sectors often see faster gains than service industries due to their unique needs and flexibility. A favorable regulatory environment enables quicker innovation and adaptation, boosting productivity. If your industry embraces AI, automation, and data analytics early, you’ll likely experience higher efficiency and output. Capital investment also plays a vital role—more funds mean better technology integration. Additionally, exposure to global markets introduces both opportunities and challenges that can impact productivity. Understanding these industry-specific factors helps you recognize why some sectors advance faster with AI, while others lag behind despite technological potential.
Measuring True Productivity Improvements
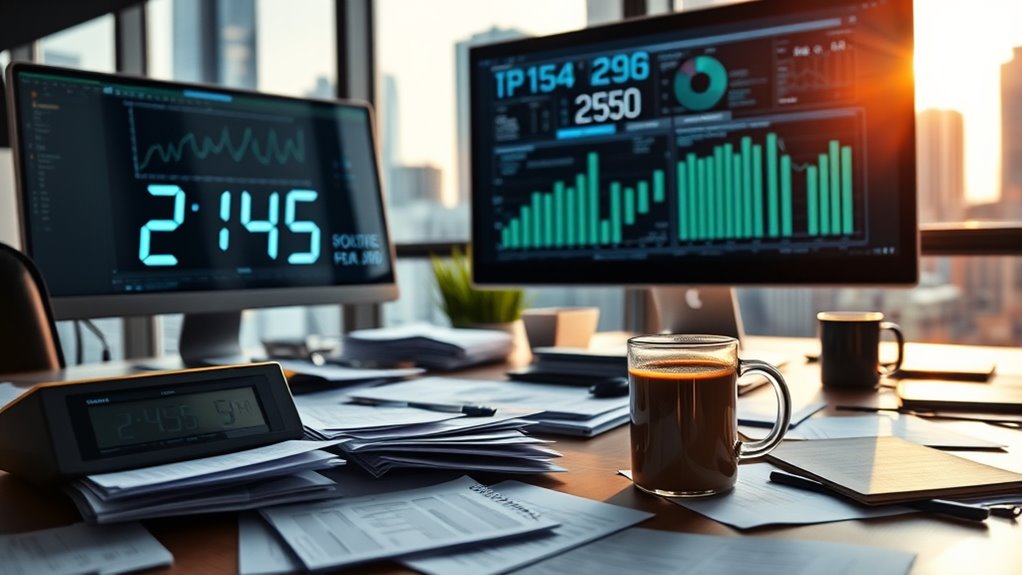
Measuring true productivity improvements requires more than just tracking output; it involves using accurate methods to assess how efficiently work gets done. To do this effectively, you need a mix of quantitative and qualitative data. First, imagine monitoring input-output ratios, like tasks completed per hour, giving you a clear view of efficiency. Second, think about using time-tracking software that shows exactly how employees spend their time—highlighting patterns and bottlenecks. Third, consider task completion rates that reveal whether your team is meeting goals consistently. Combining these measures helps you understand performance beyond surface-level numbers. Remember, consistent measurement methods and context are crucial to accurately gauge true productivity, especially when the goal is meaningful improvement, not just more work done.
Future Outlook: Bridging the Gap Between Potential and Performance
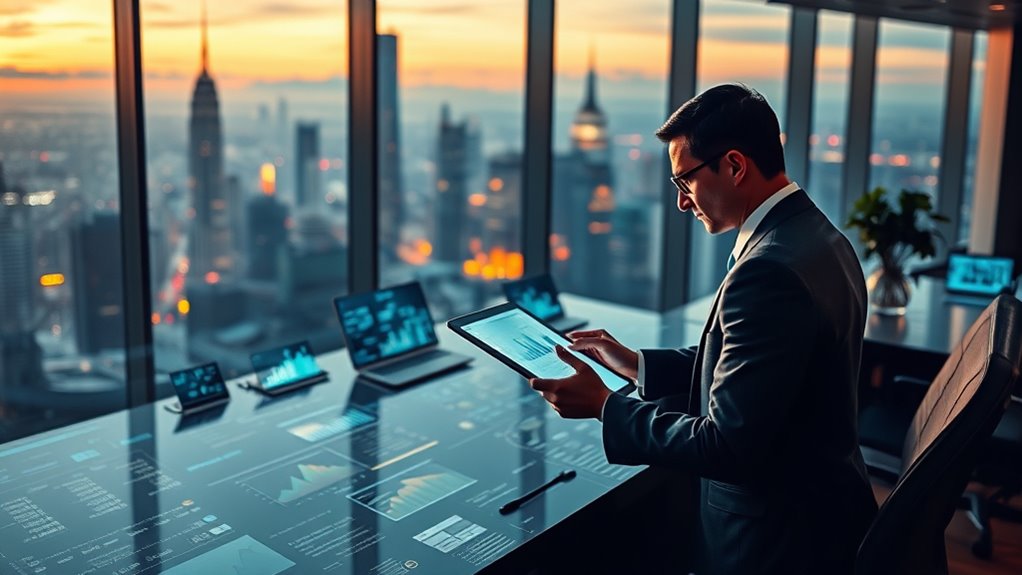
Bridging the gap between AI’s potential and actual performance requires addressing both technological and organizational hurdles. You face slow adoption, with only 20% of leaders expecting widespread employee use within a year, and nearly half of businesses lacking the talent to implement AI effectively. The adoption gap is clear—just 10% of larger companies fully integrate generative AI. Data security concerns, impacting 75% of customers, hinder AI-driven service improvements. To close this gap, organizations must focus on workforce upskilling, fostering collaboration across leadership, IT, and HR, and addressing security issues. Early adopters gain a competitive edge, but widespread success depends on strategic investments, continuous monitoring, and rebuilding trust in AI’s safety and value. Only then can AI realize its full productivity potential.
Frequently Asked Questions
Why Are AI Productivity Gains Not Visible in All Industries?
You notice AI productivity gains aren’t visible everywhere because industry-specific factors matter. In sectors with routine tasks, like retail or finance, gains show faster. But in manufacturing or healthcare, complex workflows, regulations, and safety concerns slow progress. Plus, many companies face challenges like data quality, integration issues, and workforce readiness, which limit AI’s impact. So, while some industries benefit quickly, others need more time and resources to see real improvements.
How Does Organizational Culture Affect AI Adoption Success?
You should know that organizational culture plays a vital role in AI adoption success. When your culture aligns with strategic goals and fosters adaptability, AI integration speeds up and becomes more effective. A positive, innovative culture can boost employee engagement by up to 72%, making them more receptive to new tech. Ultimately, a strong, flexible culture acts as a catalyst, enabling smoother AI implementation and maximizing its benefits for your organization.
What Skills Are Most Critical for Ai-Driven Workforce Transformation?
You need to develop critical technical and cognitive skills for AI-driven transformation. Focus on mastering advanced problem-solving, digital literacy, and data analysis to interpret AI outputs effectively. Strengthen collaboration, communication, and emotional intelligence to work seamlessly with AI tools and teams. Cultivate creativity, resilience, and ethical judgment to adapt to rapid changes. Embracing continuous learning and self-management guarantees you stay relevant and maximize AI’s potential in your workplace.
Are There Unintended Consequences of Widespread AI Implementation?
You might not realize it, but widespread AI implementation brings unintended consequences you should consider. It can increase job insecurity, fueling anxiety and stress in your workplace. AI dependence may harm your self-esteem and lead to burnout, especially if your role feels redundant. Additionally, AI surveillance and data privacy issues can worsen your morale and mental health, creating a challenging environment where productivity alone isn’t the only concern.
How Can Small Businesses Effectively Leverage AI for Productivity?
You might think AI automatically boosts productivity, but it’s more about how you use it. Focus on one high-impact task, automate repetitive work, and leverage existing data. Engage your team to find pain points AI can fix quickly. Ironically, the key isn’t just adopting AI, but using it smartly. Train your staff and monitor competitors—small, strategic steps turn AI into a true productivity booster instead of just a shiny tool.
Conclusion
You might find it surprising that despite over 80% of executives believing AI will considerably boost productivity, real-world gains remain modest. This gap shows that technology alone isn’t enough—you need smooth integration, workforce buy-in, and realistic expectations. Until these hurdles are addressed, AI’s true potential to skyrocket productivity stays out of reach. So, stay informed and adaptable, because closing this gap could redefine how efficiently you work in the near future.